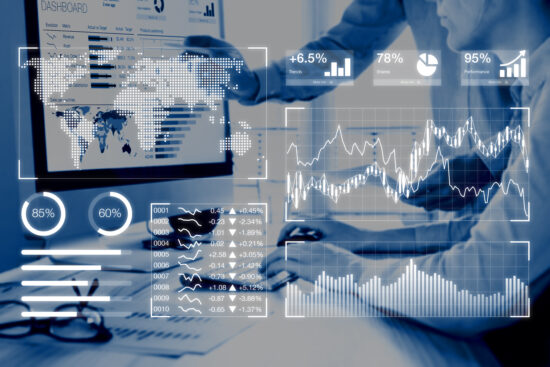
Data-based risk management in the property insurance sector
At a time when competition in the insurance industry is becoming ever fiercer and customers are becoming increasingly demanding, rapid claims processing and active risk management have become more important than ever. Using the example of property insurance, we will show you how to meet both data-based and compliance requirements with the INFONEA BI platform.
Using AI to gain a deeper understanding of claims in property insurance
From commercial liability and household insurance to policies in private health insurance: across all divisions, a sound understanding of loss causes is essential to manage risks effectively. In automobile insurance, for example, factors such as driver behavior, the type of vehicle and the claims history are included in the risk assessment and make it possible to calculate premiums specifically. Another example is homeowner’s insurance, where factors such as the building location, the risks posed by burglaries, water damage and causes of fire need to be analyzed to achieve this. The in-depth analysis of these loss drivers enables you to take preventive measures, offer needs-based policies that meet your customers’ needs more effectively, and improve the risk structure and thus the profitability in your portfolio.
How can such loss drivers be effectively analyzed? First of all, you need various types of data:
- Internal data: Claims data, contract data, policyholder master data, and other past event data.
- External data: Weather data, traffic information, property information for buildings, crime statistics and other information describing a risk to be insured in the respective context.
For one thing, such data often has to be painstakingly compiled manually. For another, a whole army of underwriters and actuaries would need to be engaged – increasingly difficult to find in view of the current shortage of skilled workers. Through the application of digital solutions based on Artificial Intelligence and business analytics, insurers can close the gaps and significantly reduce the workload on their staff:
- The merging of the relevant data can be automated.
- Identifying patterns and relationships becomes faster and more accurate.
- Risk assessment models can be developed with even greater precision.
- In dashboards, the results are prepared context-dependently so that different stakeholders (e.g., actuaries, customer service, controlling and management) obtain the information relevant to them in each case.
What does this look like in concrete terms? An example from automobile insurance
Let’s imagine that an insurance company wants to reduce the frequency of accidents in the motor vehicle sector. By conducting a data-based loss driver analysis, it can identify relevant factors that contribute to accidents. These include, for example:
- Driving behavior
- Age of the driver
- Vehicle type and other vehicle information
- Damage history
- Particularly vulnerable areas
- Hazardous weather conditions
The company can take advantage of these findings to introduce targeted prevention measures, e.g., driver training for specific groups of people or the promotion of driver assistance systems. This can reduce accidents and decrease the frequency of damage.
Visualization of damage drivers with INFONEA:
Developing technical catalogs of measures that can be taken
In the next step, technical catalogs of appropriate measures can be derived from the results obtained in this way. The measures are aimed at proactively minimizing risks and reducing loss events. This kind of catalog can include different approaches, for example:
- Preventive measures: In the automotive sector, this may include training for customers who are at increased risk of accidents or specific safety measures to improve their driving behavior. Homeowner’s insurance may include measures such as the installation of alarm systems or the promotion of fire safety precautions.
Target: Reducing loss frequency and costs. - Individualized policies & optimized underwriting: By leveraging risk analytics data, insurance companies can offer more personalized policies that are tailored to the exact needs and risk profile of their customers. This allows premiums and coverage to be customized, providing customers with tangible added value.
Target: Identify trends and patterns to offer customers tailor-made services. - Claims management: A data-based loss driver analysis also helps to optimize claims management. Automation and suggestions in claims management make for more efficient, faster claims processing.
Target: Accelerate claims settlement and reduce the workload of employees.
Transparent and compliant with trustworthy AI
There are often concerns about whether AI solutions meet regulatory and compliance requirements. There is a lack of transparency around how AI makes decisions and how these can be proven in cases of doubt.
Explainable AI (XAI) – explainable artificial intelligence provides transparency on how AI arrives at its results. This is increasingly replacing previous entirely non-transparent black-box processes. In this way, traceability and trust become the basis for AI-supported decision-making. In the loss driver analysis, it can then be determined down to the individual case level exactly which drivers contribute to which amount of damage. It is also comprehensible if the AI solution cannot explain an amount of damage because, for example, essential information is missing. This enables experts to better understand the reasons and correlations behind the findings and to substantiate the occurrence of analysis results – vis-à-vis customers, but also in audits, for example.
With INFONEA, as well as in all our projects, we use various procedures to make analysis results transparent and discussable. In doing so, we always follow the Ethics guidelines for trustworthy AI issued by the EU Commission.
Your next steps with Comma Soft and INFONEA®
Are you interested in learning more about how you can improve your claims management and risk control through loss driver analyses with INFONEA? Feel free to share your thoughts on this with us: