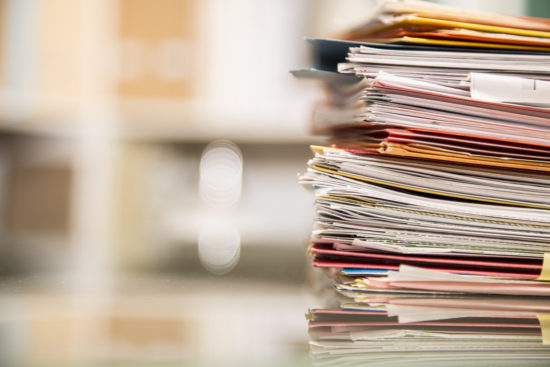
Overcoming the problem of high levels of manual effort in testing processes
When it comes to claims assessment and settlement, verifying various types of documents manually often results in high costs. In many cases, this verification is not economically viable, since either no reduction of the payment can be implemented or it is so low that the verification costs exceed this amount. In order to achieve this, information about the possible success of the inspection / the need for inspection must be made available as early as possible and used to manage the work resources in relation to a light-dark control or case prioritization.
Increasing the dark processing rate through machine learning
Higher degrees of process automation can be achieved by using modern mathematical and statistical methods (AI/machine-learning methods). Machine learning algorithms are used to identify those calculations that promise the highest possible test success in order to prioritize them for manual testing. Only the cases that definitely merit being checked are sent to the clerks, while the vast majority of invoices are processed in the dark.
Operationalization: putting AI into action
The aim is to embed the model in the corresponding operational regulation process. The first step in this process is to construct an operational and executable minimal model. Such a model can then be integrated following the corresponding rule check, for example, before it is sent to the administrator. From a technical point of view, such ML models are typically connected as microservices via REST APIs to the corresponding interfaces of the service systems. Depending on the prerequisites, integration into the testing process (call microservice and process control based on the model score) usually requires an IT implementation project.
Value contribution: what benefits can be expected from optimizing the service process?
Controlling cases in need of checking as efficiently as possible and optimizing light-dark control in the document material reduces manual effort and can result in a higher degree of automation. It is possible to achieve higher reduction sums by having the available employees check the cases in an efficient manner, which increases the overall checking result.
In principle, this form of ML-supported process automation can be applied to numerous other use cases in the field of claims auditing and claims settlement. Please contact us at any time if you are interested!