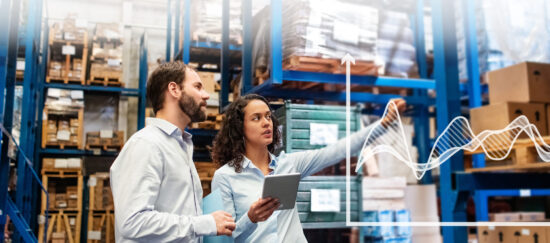
Reducing surplus and costs with the accurate planning of material requirements
For many suppliers, material planning is a complex undertaking, especially in industries that are subject to regulation. Materials must conform to the highest quality standards and often have long lead times. Original equipment manufacturers (OEMs), on the other hand, predominantly manufacture on a just-in-time basis. Many companies attempt to address this challenge by ordering more materials than they actually need. The aim here is to mitigate supply bottlenecks and delays. But what’s the consequence? Unneeded materials must be canceled, moved into storage, or disposed of. As a result, the planning process becomes more complex and excess is accumulated, creating unnecessary costs and making it more difficult to meet ESG obligations.
Accurate and forward-thinking materials sourcing ameliorates this and, accordingly, constitutes a critical factor in the success of companies in the supply chain. Machine learning (ML) based approaches are useful for making predictions for more efficient material planning and procurement.
Example: Forecasting with ML at an aerospace supplier
We have implemented ML-based forecasting with one of our customers, a global supplier to aerospace companies. They were faced with the challenge of receiving binding orders for their products with only a few weeks’ lead time, while the delivery time for raw materials for their own production was up to seven months. Forecasts for these demand quantities were based on the experience of the company’s own planning experts and on demand indications from customers. Given that customers also face the same challenges and uncertainties as their suppliers, however, their planning was more generously scaled to avoid breaching delivery deadlines and the contractual penalties that go with them. This approach resulted in ever higher inventory and capital commitment costs across the supply chain – a classic example of the so-called “bullwhip effect”. Our customer wanted to counteract this with more precise material planning.
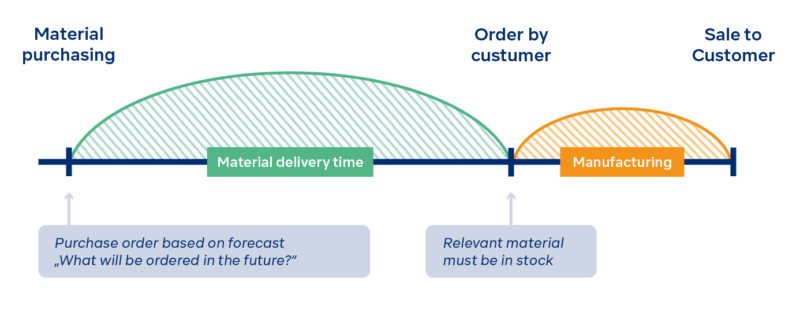
Significantly less variance in demand due to holistic analysis of order and delivery data
In collaboration with our customer, we developed a solution that leverages machine learning to analyze historical orders, taking into account external factors such as market trends and incorporating additional information such as demand indicators from customers. Taking a holistic view of all this data makes it possible to draw up more precise forecasts, e.g., when which products will be ordered in what quantities over the coming months. This allows material requirements to be accurately determined and warehouse capacities as well as production capacities to be managed more efficiently – a significant improvement on the previous purely experience-based forecasting. This makes it possible to considerably reduce deviations from the actual requirements compared to the previous material planning. This greatly reduces the risk of stock build-up or stock-outs, especially in the event of prolonged over- or underestimation of the actual demand.
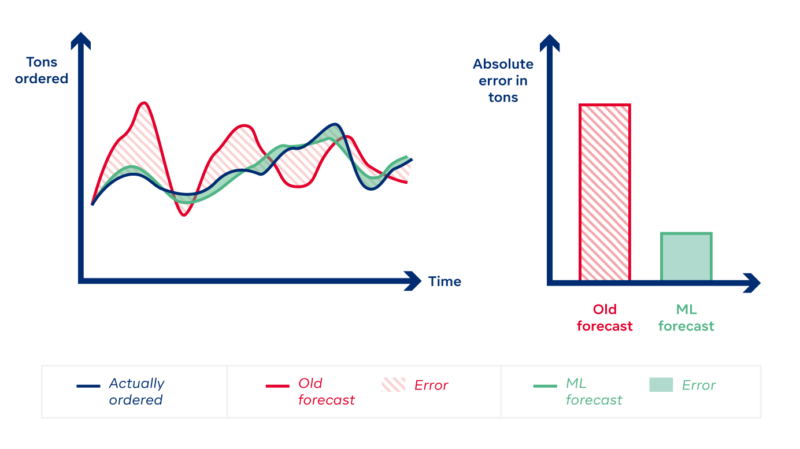
Automated processes to support planning experts
ML-based forecasting allows our customer to significantly reduce their inventory costs while ensuring that there is always enough material to fulfill their orders. At the same time, the new solution translates into an enormous increase in efficiency. Whereas planning experts previously had to manually collect and prepare demand indications, this process is now automated. Not only is this substantially quicker, the data can also be collected around the clock in real time and material requirements can always be planned up to date. This gives purchasing departments more time to focus on communication and contract negotiations with suppliers, for example.
Your next steps with Comma Soft
If you would like to discuss how machine learning can improve forecasting and planning for manufacturing companies, please feel free to get in touch.