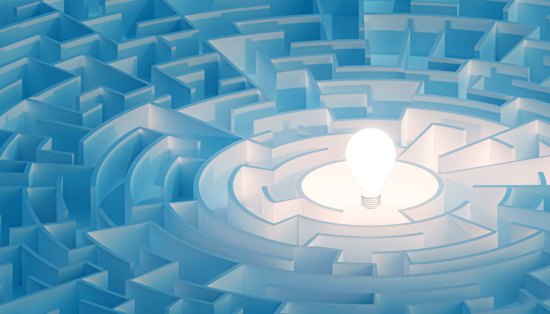
How to chat with your company’s knowledge bases
ERP, CRM, Wiki, SharePoint, BI platform: these systems, which contain company-specific and specialist information, are usually fed and updated with data by employees over a period that may span a number of years. The wealth of data and their structures grow over this time. So how can you find exactly the information you need for a specific task among millions of data entries? The good old free text search soon reaches its limits when it comes to specific questions, as it is not able to take the semantics of the texts into account – especially when different (specialist) languages are used. Employees who understand these often complex structures may depart the company for pastures new at some point and will no longer be able to provide assistance when it is needed. On a daily basis, valuable time is wasted in the pursuit of the relevant information, fruitless searches and feelings of frustration that arise from the resulting unproductivity are often the consequence. Once you have finally found what you are looking for, you usually then have to look through a page-long document to get the answers that are pertinent. Generative AI can help here. It can provide concise information and offer much more than just a list of “reading tips”.
Retrieve knowledge faster with generative AI
One strength of the new large language models is that they can find relevant information within a given context and prepare it in a way that is suitable for a specific question – and all of this can be done quite simply via a chat query.
The key to this is the so-called retrieval augmented generation (RAG). This process involves the continuous preparation of internal documents by an LLM for a semantic search and their storage as a vector representation in a so-called vector database. For a subsequent question in the chat, the most relevant document sections for answering the question are quickly picked out and added to the question as extended context for the LLM, e.g.: “Consider the following texts … when answering the above question.” This approach allows model training and fine-tuning to be decoupled from the generation of relevant documents. This improves the consistency of the answers and makes them easier to follow, since the source reference to the documents used for the answer is always available and can be called up directly by the user if required. Existing access rights to the documents can be considered, so that confidential documents remain protected.
For example, caseworkers can ask generative AI how to create an application for a new project, instead of searching the depths of databases by keyword. In reply, they will receive step-by-step instructions and similar previous project applications as references. This not only saves time, but also ensures that all relevant information is taken into account and irrelevant information can be excluded.
Other examples of information that can be used with generative AI as answers to specific questions include:
- Relevant contractual terms and conditions for various service products (e.g., insurance conditions)
- Media published by the company such as newsletters, employee magazines, etc.
- Legal texts and similar regulatory requirements
- Tenders
- Scientific research articles
- Patents
- End-user documentation for software or information from the support desk
- Standard operation procedures (SOP)
- Failure & situation reports as well as reports on FMEA (Failure mode and effects analysis)
- Fault, maintenance & repair logs
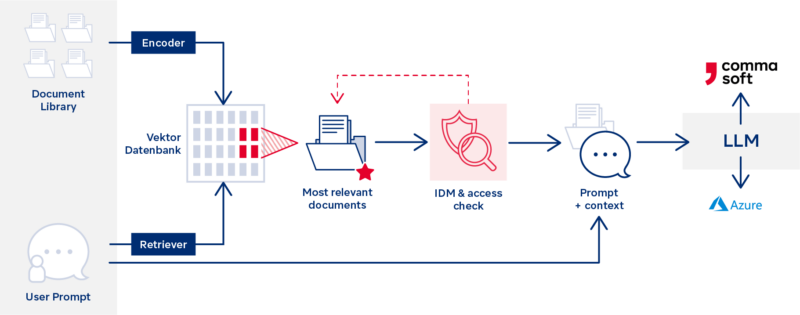
Combining language models and in-house knowledge bases
For common AI tools that extend SharePoint as plugins, for instance, usually only the content for GenAI-generated answers that is in that specific application is usable. For the connection of further external sources, elaborate customizing is usually necessary.
In the approach to knowledge management with GenAI presented here, consideration is given from the outset to the fact that multiple sources should be searchable – because the scattered storage of information in different silos represents the basic problem in retrieval. A technical solution to this is to couple generative AI with the various knowledge bases. This requires knowledge of natural language processing (NLP), data science, and machine learning operations (MLOps), as well as IT infrastructure and IT security, among other things. If a company possesses these capabilities, it can integrate its knowledge bases with the language model of a third-party provider, e.g., ChatGPT. Additionally, the GenAI solution can also be integrated into familiar user interfaces such as SharePoint or the intranet, so that employees can work with their specialist applications as normal and do not have to switch to the internet browser for every query.
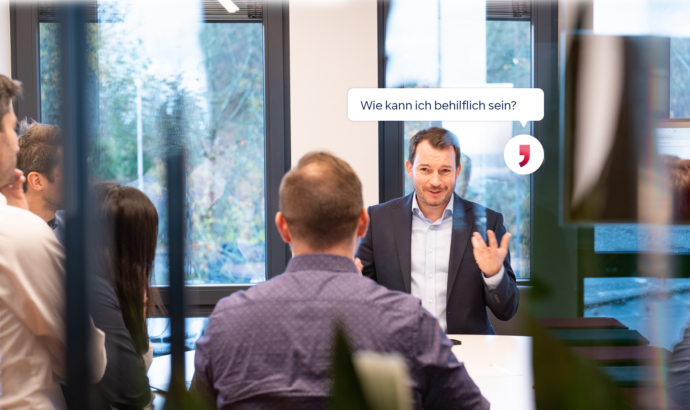
Safe with in-house LLM
Not every company wants to use proprietary LLM services such as ChatGPT – especially in a regulated environment or with special compliance requirements. If this is the case for you, we recommend an in-house LLM where you decide where it is hosted and what happens to the processed data.
Boost your knowledge management capabilities with Generative AI together with Comma Soft
If you would like to use generative AI – whether a company-owned or a market-standard one – to support your knowledge management, we will be happy to assist you: in an advisory capacity, in the implementation of individual sub-steps or the entire process, whatever suits your requirements. Please feel free to contact us for a first non-binding consultation.