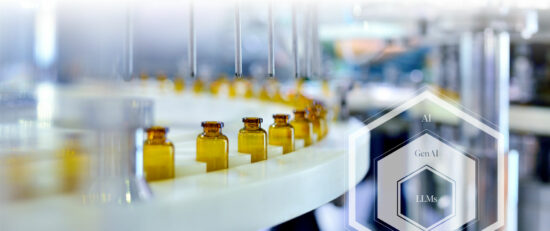
How GenAI impacts the Pharma and Life Science Sector
This whitepaper offers decision-makers in the pharma and life science industry comprehensive insights into the transformative power of GenAI across the whole pharmaceutical value chain. It reflects in which areas of application large language models (LLMs) can add value and discusses the benefits as well as the challenges behind this technology.
Generative artificial intelligence (GenAI)—resp. large language models (LLMs) as a subcategory of AI—have the potential to fundamentally change the pharma and life science industry and to redefine the way biopharma companies develop, produce, and market novel treatments. LLMs can be utilized across the entire pharma value chain, including research & development (R&D), operations, commercial and medical affairs, and corporate functions. In these fields, this technology has the potential to boost the output quality and productivity and is able to democratize knowledge and thus drive innovation.
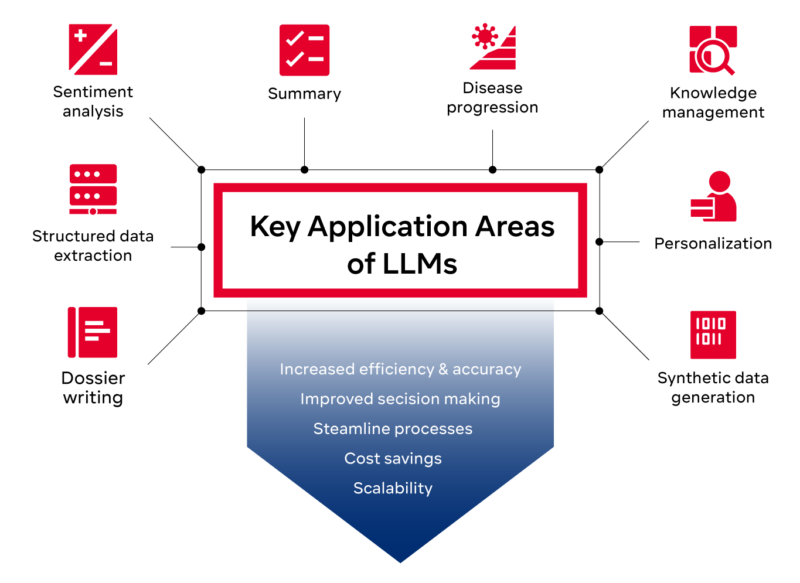
However, implementing GenAI in a highly regulated industry presents considerable challenges. Successful deployment not only requires strategic application and scaling, but also effective management. This necessitates a strategic alliance of technology and business strategy, tailored to an organization’s unique circumstances.
In order to avoid the risk of losing market share in this rapidly evolving landscape, it is essential for companies to take proactive steps towards adopting these technologies.
In the following, we examine three key application areas of LLMs:
Through these examples, you will benefit from our practical experience in developing and implementing GenAI solutions and be able to shorten your path to making a part of your business operations.
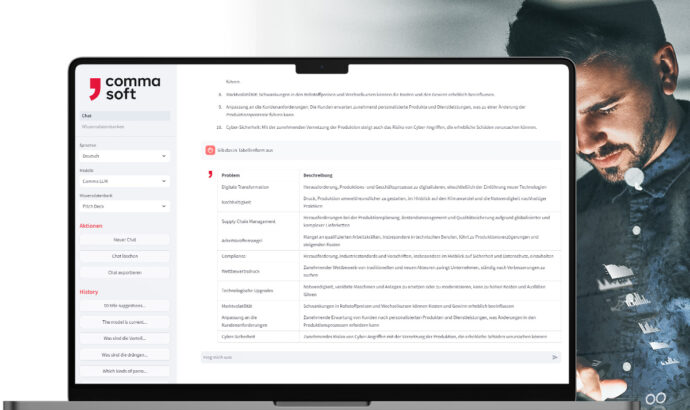
Alan: Your secure GenAI
With Alan, the Comma LLM, you use your company’s own GenAI service – ready to use in a European or German cloud or on-premises. Here you can integrate your own information and control what happens to your data and how results are generated.
Side note on the terminology
Large language model—LLM—generative AI—prompting: the terminology might be confusing. Those who are interested in digging deeper can do so here.
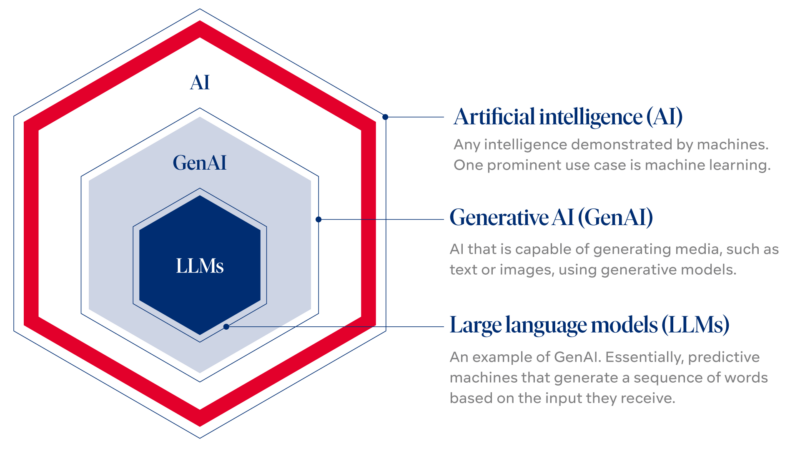
-
AI refers to any intelligence demonstrated by machines. Colloquially, the term “artificial intelligence” is applied when a machine mimics “cognitive” functions that humans associate with other human minds, such as “learning” and “problem solving”. It is a field of study in computer science that develops and studies intelligent machines. One very prominent use case of modern artificial intelligence is machine learning, e.g. learning from huge amounts of data how to label images or predict time series.
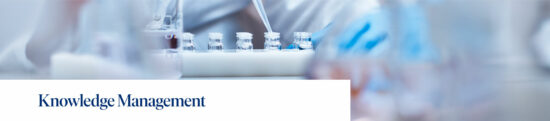
Managing knowledge with LLMs
The pharmaceutical industry is a data-intensive industry. Therefore, knowledge management plays an integral role, particularly in the context of standard operating procedures (SOPs) which demand the consumption of a myriad of documents by multiple stakeholders. This often involves manual reviews of voluminous procedural documents, technical reports, and research findings. Each role, each phase of research, production, or testing has its own set of protocols and procedures that must be meticulously followed.
Standard operating procedures: from time-consuming search to efficiency and scalability
The current process of knowledge management in the pharmaceutical industry is mostly paper intensive and associated with a lot of manual effort. SOPs are stored in document repositories, and the onus is on the individual to find the relevant information from the pile of documents. In addition, the technical language used in these documents often creates a barrier for non-technical stakeholders such as colleagues in cross-departmental projects, leading to communication gaps.
Overcoming the challenges of managing knowledge in pharma
The current knowledge management system presents several challenges. The most glaring is inefficiency—the process of sifting through hundreds of documents is time consuming and can feel burdensome. Misinterpretation of information due to language complexities or specific jargon can lead to procedural errors, impacting the organization’s overall productivity as well as entailing the risk of legal consequences.
Another challenge is the inability to scale. As an organization grows, the volume of SOPs and other documents increases dramatically. As a result, the time and resources required to manage and access this knowledge increase. This current situation often creates bottlenecks that slow down the decision-making process, which may lead to competitors gaining the upper hand in the race for innovations and patents.
Opportunities & possibilities brought by using LLMs in the field of knowledge management
LLMs can revolutionize the way we handle knowledge management and SOPs in the pharmaceutical industry. As these models can read, evaluate, and generate text in a human-like manner, this makes them ideal for managing large amounts of information. They can be trained to read SOPs, extract relevant information, and present it in response to specific queries. The vision of pharmaceutical companies might be to create a democratized AI environment where any employee, regardless of role or technical acumen, can access and understand SOPs. The LLM would serve as an intelligent assistant, helping each stakeholder to communicate effectively and thereby increasing the overall efficiency of the organization. Instead of having to manually search through multiple documents, employees can simply ask the LLM a question via a prompt. The LLM, with the help of RAG, can process the query, search the database of SOPs, and provide the answer directly, citing the relevant parts of the SOPs.
Benefits of managing knowledge with LLMs
- Scalability: An LLM can serve thousands of employees simultaneously, answering questions related to different roles, phases, and procedures.
- Bridging communication gaps: The LLM’s ability to interpret and generate text in the user’s language makes it a powerful tool for bridging communication gaps. It can decode technical jargon and explain it in simple terms, fostering better understanding across different teams.
- Cost savings: Finally, the democratization of AI allows any employee to use and benefit from LLMs. This can lead to cost savings by significantly reducing the time spent searching for and understanding SOPs.
- Reduced potential for errors: It also improves the accuracy of the information provided, reducing the potential for errors.
Challenges of using LLMs for knowledge management
- Security: Companies using an LLM must prevent internal information from leaking out. As many available GPT tools run in US-based clouds or have untransparent data protection measures, these tools are not suitable. Especially in regulated sectors, companies should ensure that the LLM they want to use fulfills their need of a secure, regulation-conforming and controllable LLM.
- Updates: If implementing an in-house GenAI solution, companies have to make sure that the LLM is regularly trained with the latest information (e.g. new regulations) resp. gets access to this data via RAG.
- Language: Common GenAI tools are only useful if their users know how to prompt effectively. To ensure that everyone is able to profit from the LLM, it should be possible to use it in an easy way, i.e. in the native language of the respective user, by designing system prompts accordingly.
- Business context: Standard GenAI market solutions are not trained with technical terms from the pharmaceutical industry. Companies should check if the LLM they want to implement is trained for their specific wording resp. if it is possible to train the LLM with little effort.
Further use cases for knowledge management with LLMs
Research and development (R&D):
Researchers can use LLMs to quickly access SOPs, papers and studies related to various phases of drug development, thus speeding up the R&D process.
Quality assurance (QA):
QA teams can use LLMs to ensure compliance and regulatory standards, reducing the risk of non-compliance.
Production:
LLMs can assist production teams in understanding SOPs, specifications, and documentations for various processes, thereby minimizing production errors.
Training and onboarding:
New employees can use LLMs to quickly familiarize themselves with compliance requirements and specialized knowledge of their division relevant to their roles, reducing the time and effort spent on training and onboarding.
Compliance management:
Regulatory teams can use LLMs to ensure that the company’s practices align with the latest national and international regulations by querying the model about specific regulatory standards.
What to reach by managing knowledge with LLMs
The use of LLMs to manage SOPs and many more sensitive processes has the potential to bring about a paradigm shift in the pharmaceutical industry’s knowledge management. By making knowledge easily accessible and understandable, LLMs can increase efficiency, improve communication and lead to significant cost savings.
The democratization of AI with LLMs ushers in a new era in which every employee, regardless of their role or technical acumen, can benefit from AI, ultimately leading to a more efficient and productive pharmaceutical industry.
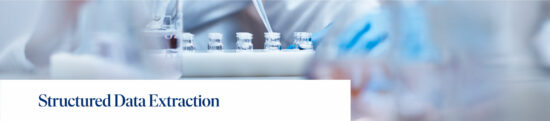
LLMs in structured data extraction
The pharmaceutical industry is rich in data and information from various sources such as protocols, clinical trials, electronic health records and more. The data is diverse and complex, with a lot of implied knowledge and abbreviations that are specific to this field. However, much of this data is unstructured and therefore underutilized in decision making and research.
From wild data growth to data maturity
As ever more data is accumulated in pharma companies, and often is stored in historically grown and mostly isolated applications, the need to manage this data efficiently becomes urgent. Using LLMs, companies get the opportunity to streamline all their data by extracting structured information from unstructured sources and gain data maturity. With LLM-based data extraction, it becomes possible to gain valuable insights and improve decision making across processes and divisions in a much more efficient and faster way than ever before.
Overcoming the challenges of extracting data
One of the major challenges is the extraction of relevant information from the vast amount of available structured and unstructured data. Manual data extraction is time-consuming, requires substantial effort, and is prone to errors. Moreover, the information is often written in many different ways or different terms are used, which makes it difficult to find the relevant information.
What is more, a big part of the data may be stored isolated in various systems and different versions. When it comes to data extraction, there is a risk that outdated data is used as it is often unclear which of the existing versions is the latest and whether all relevant information has been updated in the latest version.
Opportunities & possibilities brought by using LLMs for data extraction
LLMs present a promising solution to these challenges. They are capable of evaluating the context and semantics of a given text, making them exceptionally skilled at extracting data. They can automate the data extraction process, reduce errors, identify outdated data versions, and uncover insights from the latest data. This allows for better organization and analysis of the data and enables the organization to identify patterns and relationships that matter. LLMs are able to identify and anayze domain-specific abbreviations and implied knowledge in various documents, enhancing the accuracy and reliability of the data.
Benefits of data extraction with LLMs
- Efficiency: LLMs can extract and analyze different kinds of data from various sources with high accuracy and within seconds.
- Scalability: LLMs are able to handle large volumes of data easily. As the amount of data is permanently growing, its extraction can be scaled up with LLMs instantaneously.
- FAIRification of data: FAIR data (Findable, Accessible, Interoperable, and Re-usable) is an important step toward a data-driven company. LLMs are able to extract relevant metadata from structured and unstructured sources, thereby helping to overcome the first hurdle by making data findable through sufficient metadata. In addition, LLMs can also make an important contribution to the remaining FAIR dimensions.
- Standardization: LLMs can harmonize the information and data in documents, which reduces inconsistencies and subjectivity in the data. This facilitates a better communication and understanding of data throughout the whole company, as the standardization into a universal language becomes possible.
Challenges of using LLMs for knowledge management
- Cost transparency: Many GenAI tools offer pay-by-token subscriptions, which may end up increasing costs. Using an LLM, companies should be aware of the pitfalls of the subscription model and choose an LLM which meets their budget, e.g. by choosing a subscription without user restrictions.
- APIs: Using an LLM for data extraction means companies will have to connect it with all relevant applications the data is stored in. Not all GenAI tools support all APIs. It is necessary to make sure that the integration is possible.
- Access: To be able to extract data from sources, the LLM has to be able to access these sources in the first place. It is therefore not only important to have technical ways of accessing all data, but also to have governance and/or rights and roles concepts in place to make sure that users are only able to access extracted information from sources they are allowed to see.
Further use cases for extracting data with LLMs
Patient data:
LLMs can be used to extract and categorize patient information from electronic health records, as well as recover missing patient data from unstructured text such as discharge summaries.
Competitive intelligence:
By analyzing news articles, blog posts, and other relevant content, LLMs can uncover patterns and trends, such as changes in the competitive landscape, pricing or patient preferences that may impact a business’s industry or target market. This information can be used for strategic decision-making and help capitalizing on new opportunities.
Regulations:
LLMs can be used to extract regulations for one specific purpose from thousands of pages of regulatory texts, expediting and enabling compliance.
Post-market surveillance & pharmacovigilance:
The detection, assessment, understanding and prevention of adverse effects or any other medicine-related problems and the general post-market surveillance is not only required by regulations but also an important source of information for the pharmaceutical industry. Although some structured data is recorded by special systems, there are also public sources, such as social media or forum/blog posts, as well as unstructured internal documentation. LLMs can create structured data from these sources very efficiently, thus speeding up the process and increasing quality through data that was previously unusable or difficult to use.
What to reach by structured data extraction with LLMs
LLMs hold significant potential for structured data extraction in the pharma and life science industry. Their ability to automate the extraction process and uncover complex insights from the data can greatly enhance the industry’s ability to innovate and make informed decisions.
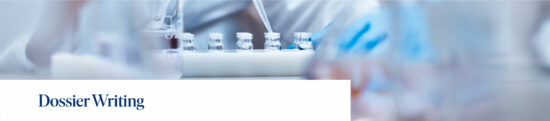
LLMs in dossier writing
In the pharma industry, one of the most critical tasks is the creation of comprehensive dossiers used for, among others, clinical study approvals, clinical and preclinical protocols, research protocols, and audience-specific reports. These complex documents are currently created by teams of experts who manually gather, interpret, and organize vast amounts of data—a process that is time-consuming, resource-intensive, difficult to standardize and/or error-prone.
Reaching out for more standardization and consistency
The process of dossier writing is also fraught with inefficiencies due to the sheer volume of data and the stringent requirements of different regulatory bodies and audiences. Moreover, it is difficult or even impossible to maintain uniform standards at all times, especially in large organizations with a large number of distributed departments staffed by many specialists. This is where LLMs can help.
Overcoming the challenges of dossier writing
The manual process of dossier creation presents numerous challenges. Time and resource consumption are substantial, leading to increased costs and delayed submissions. The risk of inconsistencies and errors due to human involvement is high, potentially impacting the acceptance rate of submissions. Hence, there is a high need for quality assurance to ensure the accuracy and completeness of information. This is particularly challenging when hundreds of people in different teams distributed around the world have to produce documents with a uniform appearance and standardized language.
It is almost impossible, even with the assistance of comprehensive search tools and document management systems, to always be aware of and incorporate all potentially relevant data sources, especially when it would be highly beneficial to track regular changes. It is not always possible to instantly react to source modifications when the work has to be carried out by humans.
Opportunities & possibilities brought by using LLMs for dossier writing
Generative AI and LLMs can effectively address these challenges by automating the dossier writing process. These models can analyze vast amounts of data and generate accurate, comprehensive documents tailored to specific requirements, significantly reducing the time and resources required. Organizations can expect a more strategic approach to their compliance and communication processes.
It is important to note that experts will, however, still play a crucial role in this process, but their focus will shift from generating content to reviewing, criticizing, and finalizing content. This ensures that their specialist expertise is put to much better use and, in addition to increased efficiency, also leads to much higher quality, as the experts can concentrate on the really critical aspects.
Benefits of writing dossiers with LLMs
- Efficiency: AI automation with LLMs can significantly reduce the time and resources required for dossier creation. Furthermore, LLMs increase the effectiveness of communication with different interest groups without the need for time-consuming manual rewording of documents. All of this leads to cost savings and a faster time to market.
- Accuracy: By using LLMs, human errors can be minimized, and more sources can be included, which, combined with the expertise of human experts, leads to better quality and more consistent dossiers.
- Application success: LLMs can analyze dossiers, identify critical passages and predict the success rate of applications, allowing companies to plan strategically and proactively address potential issues before submission.
- Capacity: LLMs, unlike human experts with limited capacity, can be scaled to handle large volumes of data and work on an almost unlimited number of tasks, enabling the production of high-quality dossiers even in areas previously limited by resource constraints. This not only broadens the scope of dossier creation but also ensures consistency across all sectors in the organization.
Challenges of using LLMs for dossier writing
- Implementation: LLMs have to be integrated into existing systems and must be trained with relevant data. It is crucial to ensure that the AI system can handle the volume and complexity of the data involved and is also trained on and correctly using the required technical language for the respective application.
- Security: While GenAI offers promising solutions, it is vital to be aware of potential pitfalls. To avoid them, companies should ensure that their LLM fulfills the data security and privacy requirements, avoids potential ethical issues related to AI use, and maintains transparency in the AI’s decision-making process.
- GenAI literacy: Identifying critical passages or predicting the success rate of submissions is a potentially valuable strategic tool. Companies should train their employees so they are able to evaluate the quality of the results.
Further use cases for writing dossiers with LLMs
Clinical trials dossier:
LLMs could be used to compile a comprehensive dossier for a clinical trial, analyzing data from various trial phases, customizing the structure to meet specific regulatory requirements, and identifying potential red flags that could complicate the approval process.
Protocol generation:
LLMs could generate protocols for clinical and preclinical phases, analyzing the trial design and results to produce accurate, clear, and concise protocols tailored to different target groups.
Risk assessment:
During dossier creation, the LLM can assess critical parts/risks from the input data, point them out, and provide a predictive success rate for the intended goal (e.g., the submission to regulatory bodies), enabling organizations to plan strategically and address potential issues proactively.
Reports on demand:
Creating comprehensive reports becomes even more complex when these reports have to be created for different target audiences. LLMs can create target-group-specific, focused reports from the original data at any time, virtually in real time.
What to reach by writing dossiers with LLMs
Using LLMs for automatic dossier writing has the potential to revolutionize the pharma and life science industry, driving efficiency, accuracy, and strategic decision-making to new levels. This technology is not just an option for the future—it is a practical solution for the present, ready to be embraced for the significant benefits it can deliver.
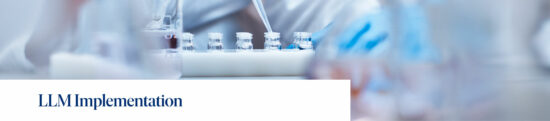
5 Guiding principles for LLM Implementation
As seen above, pharmaceutical and life science companies can benefit a lot from LLMs but have to face some challenges concerning the implementation. The following five guiding principles provide assistance in overcoming these obstacles.
1. Define the use case
While LLMs in their current form are clearly a breakthrough innovation and will lead to major changes across all sectors and areas of life, it is crucial to separate the hype from its actual potential. Therefore, it must be evaluated for each individual use case whether it will benefit from the specific capabilities of an LLM. While LLMs are a powerful technology, they are not a one-fits-all solution. As set out above, they will offer a lot of value for some use cases. For other use cases, different well-established and well-tested machine learning technologies, such as classification or clustering approaches, may be a better choice, e.g. for the deduplication of data or for optimizing processes in test runs. It is essential to weigh up the costs and benefits for each case.
2. Choose the appropriate large language model
When it comes to selecting the right large language model for your organization, several factors come into play, and the choice is not always straightforward. Firstly, the hosting environment of the model must be decided. Here, the choice is typically between on-premises or cloud-based models. On-premises solutions offer enhanced control and security, making them suitable for organizations with stringent data protection requirements, as they allow for the data to remain within the organization’s own secure environment. On the other hand, cloud-based models offer scalability and flexibility, often at a lower cost, making them an attractive option for organizations seeking cost-effectiveness and ease of scale. As with all cloud-based services, depending on the use case, it might also be important where and how exactly the cloud service is hosted.
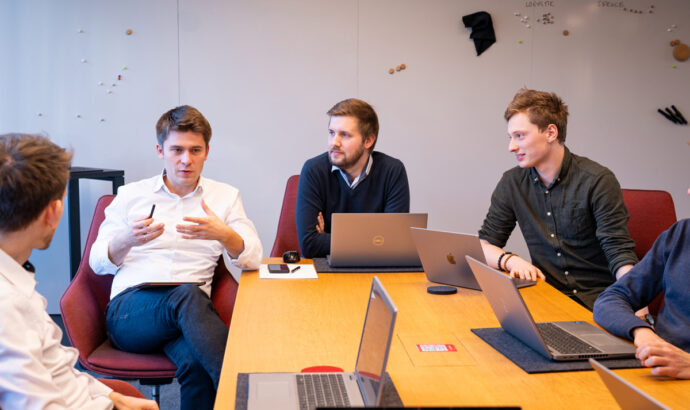
LLM on-premises
Implementing generative AI as an as-a-service solution, hosting the LLM yourself or in the cloud? In our GenAI Tech Talk, our experts provide answers to the 10 most frequently asked questions from CDOs and CIOs about hosting LLM solutions.
Next, there might be models which are already specifically trained or fine-tuned for the specific use case (as opposed to the very broadly trained models most commonly used right now). In this context, it is also important to understand that several models are often used together to cover a specific use case. For example, the real-time scoring of results provided by a very large model might be achieved by a separate smaller model to cut cost.
3. Prepare your data landscape
LLMs will perform context-specific activities at a granular level. This requires custom domain-specific data, semantics, knowledge, and methodologies. Hence, a strategic and disciplined approach to acquiring, growing, refining, safeguarding, and deploying data is required. Effective data governance and MLOps are fundamental to ensure the data is managed properly. This includes maintaining data quality, ensuring data privacy and security, and implementing data management processes that are in line with regulatory standards. Ethical concerns, such as biases in training data, have to be addressed as well. Custom developments such as ontologies can provide structure and context to data, help organizing it and make it easier to retrieve and use.
Furthermore, it is important to note that data literacy and awareness are key. Staff at each step of the value chain should be trained on how to use GenAI/the LLM in their work. This ensures that they are equipped with the necessary skills to effectively leverage this technology and contribute to the successful implementation and operation of the LLM in the organization.
4. Set up compliance and security measures
Compliance with legal regulations on data protection and IT security is of great importance for the regulated pharma and life science sector. Large amounts of data are required to train LLMs, of which some may contain sensitive data. It is necessary to ensure that the models comply with the applicable data protection laws and regulations as well as with internal compliance rules. Considering compliance or regulatory challenges, the use of an on-premises solution can prove to be beneficial.
Furthermore, data protection measures such as identity and access management (IAM) or zero trust ensure that only authorized personnel have access to certain data. This is essential to maintain the integrity and confidentiality of the data.
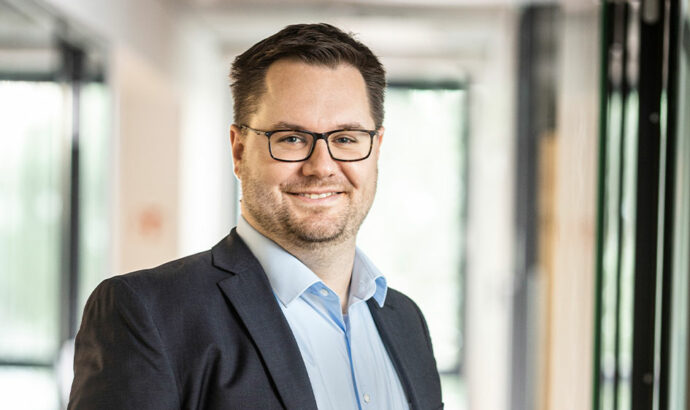
Zero Trust: what does it mean?
What characterizes a good Zero Trust architecture? Which relevant pillars should you focus on when implementing it in your company? Dr. Jan Ciupka has compiled the most important information about this cyber security concept for you.
5. Ensure your organization’s GenAI readiness
Besides the technological requirements, it is also important to make sure that mindset and culture are ready für the use of LLMs and GenAI solutions. On the one hand, this means that employees are trained to use LLMs, to validate the results and to correct them. On the other hand, it is necessary for ongoing innovation that employees identify LLM use cases and process improvements themselves. For this purpose, they have to understand the possibilities and limits of the technology and be aware of the possibilities and risks LLMs entail. Last but not least, LLM or GenAI readiness goes hand in hand with data literacy—because the quality of LLM-generated content depends on the data quality, which in turn begins with correct data input and data management.
Do you have any questions?
If you are interested in Generative AI and Large Language Models and would like to discuss the topic further, please contact Dr. Benedikt Reiz and his colleagues. We look forward to hearing from you!