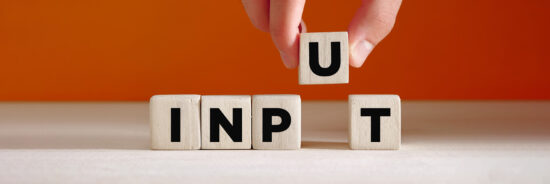
Using AI and machine learning to handle customer concerns end-to-end in digital form
More and more insurers are creating a digital service experience for their customers that is not tied to time or location: they offer policyholders various digital channels and provide contact options at a wide range of touchpoints. Contact forms on a website, chat facilities, messenger services and apps now supplement emails and the enduring contact options of telephone, fax or a letter in the post. However, this also results in a patchwork of input channels that is often difficult to reconcile at first glance. Insurance companies are faced with the challenge of immediately assigning all these messages to the correct departments, case handlers and business transactions. If this takes too long or is done incorrectly, the customer experience quickly falls by the wayside – and, in the worst case scenario, policyholders may move to the competition. Most insurance companies are already using input management systems to prevent this, with the aim of processing customer concerns digitally from start to finish and therefore more quickly and efficiently. Nevertheless, the desired success does not always come about as a result.
Example: correct GeVo assignment shortens waiting times for policyholders
When it comes to capturing, identifying and assigning incoming messages, keyword-based methods quickly reach their limits. The error rate is high and scanned documents that have been arranged end to end are not automatically separated. This increases the amount of manual work involved in re-sorting the documents, time is lost and the policyholders have to wait longer for information. Better results – and hence faster processes – can be achieved with intelligent input management that features integrated AI & machine learning components. This input management system recognizes and classifies hundreds of document types or business transactions with near-perfect accuracy. It is based on input data, both historical and new, which are continuously being added and used to train an AI model. Such deep learning-based language models classify incoming messages based on data such as insured or policy number, address, name, etc. In contrast to keyword methods, however, they can also be used to recognize natural language, unstructured data, e.g., certain word combinations that indicate the sender’s mood, the type and urgency of the request, and much more. This means that customer inquiries are recorded much more precisely, can be prioritized, and can be assigned to the right case handlers and business transactions.
Target image: maximum automation and assistance in processing customer concerns
Artificial intelligence can be used to improve and accelerate numerous sub-processes in incoming mail processing. This starts with improving the recognition quality of OCR/ICR components in the scanning line and continues through page and document separation to efficiently extracting specialist data for further use in downstream processes. If, for example, an inbound customer request is identified as a change of bank details, the next step is automatically extracting the new IBAN and updating it in the master data. This even enables fully automated dark processing for any number of customer concerns. This frees case handlers from having to perform manual, repetitive tasks that do not require specialist knowledge. Numerous product providers for input management solutions now offer suitable interfaces for connecting to such AI modules. In order to create synergy effects, it is useful to take a holistic view beyond incoming letters in the post, taking into account all the relevant downstream processes. It is therefore worthwhile to consolidate all input management system requirements and harmonize them with the rest of the IT strategy in order to avoid additional work and disruptions in processes.
Creating synergies: how does efficient input management work through to the downstream processes?
Information extracted in a targeted manner helps with subsequent processing of customer concerns in numerous follow-up processes. Typical examples include:
- Fully automated processing of incoming invoices (dark processing)
- Increase of dark processing in claim verification and claims settlement
- Improvements in fraud detection
- Automation and smart assistants in underwriting and risk assessment
- Automated removal of personal data in accordance with GDPR
- Automated compliance with legal retention and deletion periods
This means that an input management system optimized by AI has an impact deep into the insurer’s downstream processes and helps to increase the automation and dark processing rate significantly. This directly helps to reduce processing times and operating costs, and also has an effect on the satisfaction of the unburdened employees, which in turn has a noticeable effect on the quality of the service they provide.
The next step: how to optimize your input management with Comma Soft
How input management is integrated into existing processes and the modus operandi are unique to each insurer. Another factor is the different starting points: should improvements be made to an existing management system, should a new one be rolled out or should an in-house solution be developed? We are happy to help our customers think through all of these scenarios. Whether you want to optimize an existing system or introduce a new one, we recommend compiling an inventory beforehand, which we can work on with you before we embark on rolling out a tailored solution. In the process, we will answer the following questions together, among others:
- Target image and vision: what do you want your multi-channel customer communications to look like in the future?
- What are the requirements and target image for the functional and technical design?
- Is an external vendor needed for scan path and OCR software?
- Do you want to connect to Microsoft Exchange or other email servers?
- Into which other management systems should the results be fed?
- Which processes and workflows will be initiated after input management?
- How will employee capacities and skills flow into GeVo processing?
- What is the quality of existing training data for potential AI components?
This is followed by designing and implementing the solution that best meets your identified requirements. We always keep your corporate strategy in mind, as well as current and upcoming challenges.
If you would like to discuss how you can modernise your inbox in a future-proof way and increase the data quality in follow-up processes, please feel free to talk to Dr Valentin Kemper: you can contact him here.