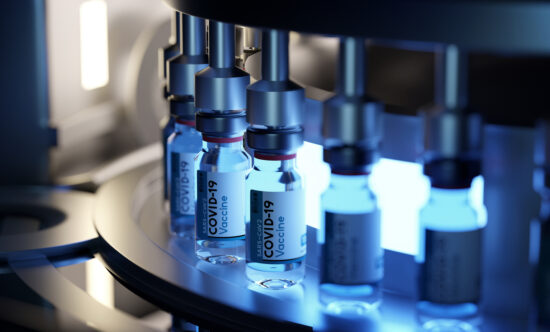
Reducing test runs and avoiding production downtimes
The new drug is developed, and production can start – if only it weren’t for the regulatory authorities and their GxP requirements! It is not only the product itself, but also the packaging that must meet stability and integrity criteria of the pharmaceutical industry. There is a requirement to provide evidence that the packaging is in compliance with the relevant standards, while at the same time meeting internal requirements in terms of quality, cost efficiency and brand representation. And while the preliminary tests to determine the optimum packing parameters are being run, the production line itself often grinds to a halt. Indeed, even if the experts at the machines are very experienced and have a solid instinct for a parameter range that makes sense to test, several rounds of testing often need to be run first. There is simply too much complexity involved in the parameter space and parameter dependencies, as well as numerous combinations of packing materials, each of which is optimized on an individual basis. We had to ask the question of whether there is a faster, simpler and more sustainable way of working than through trial and error.
Reliable data rather than a gut feeling
As a matter of fact, extensive data from the Production department and from previous experiments that have already been completed usually does already exist in the pharmaceutical production sector. This data can be used to generate a set of rules to determine the correct and optimum settings for unknown packaging materials, instead of having to rely on cumbersome measurements to find them. The challenge here lies in optimizing those parameters in the high-dimensional space that also have the greatest (direct) impact on the subsequent quality, and not getting bogged by those that have little or even no impact. The solution to this may look something like this: first, we review the plausibility and usability of the data together with the Engineering & Technology department and the Production department, as well as a number of other experts. The next step involves finding correlations, variances, gaps, and anomalies. Classic statistical and data analysis methods are well suited for this purpose. Nevertheless, the highly complex, multidimensional data generated in pharmaceutical production is often quite difficult to visualize and therefore cannot really be evaluated. Here again, a manual analysis would have to be connected. This is where machine learning can help. It can shed some light on parameters via feature importance, the influence of feature engineering, and prediction quality as a function of various packaging material properties.
These insights would make it possible to identify the main drivers of influence and to specify the parameters in which a large degree of variance is still hidden. Instead of fine-tuning based on gut feeling, this yields valid main parameters that only need to be confirmed.
Digital Twins
Faster drug approval, personalised treatment, improved prevention: digital twins hold enormous potential for research and healthcare.
Find out here in which fields of application they are used, how regulatory and compliance hurdles can be overcome with federated learning and what role a data & AI strategy plays in this.
Machine learning as a route out of the machine learning black box
Machine learning is the lever that can be used to open the door to the unknown parameters. That said, ML solutions are generally very complex, require a great deal of maintenance, and the decisions made using ML models are difficult for regulatory authorities to follow. Such an approach would not be sustainable and would only postpone, rather than reduce, the regulatory burden. The solution lies in the fact that machine learning is ultimately no longer needed. Ideally, a clearly comprehensible formula will be derived on the basis of the most important parameters and variables identified by machine learning, which will then be used in data-informed experiments to confirm or test a very small parameter space. This eliminates the need for resource-intensive, permanent ML projects. Simultaneously, the formula obtained is explicable and so satisfies the regulatory requirements.
Check requirements
To make this solution work, a pharmaceutical company must already have attained a certain level in “digital mindset” terms. This is because it is only when adequate relevant data is available, processed, and sampled very evenly across the state space that correlations and dependencies can be revealed through classical statistics and machine learning approaches. If this prerequisite is fulfilled, monotonous processes can be avoided, decisions can be made in a transparent and data-based way, and production processes can be set up more efficiently.
What’s more, the universality of the solution also allows it to be easily adapted to other production lines in the future, as only a very small number of selected measuring points need to be recorded. This combination of classic expertise and digitization is the key to sustainable optimization.
Do you already meet the requirements for this type of predication of production parameters or are you currently laying the foundations for this? Reach out to our pharmaceutical expert Dr. Henning Dickten and let’s find out together!