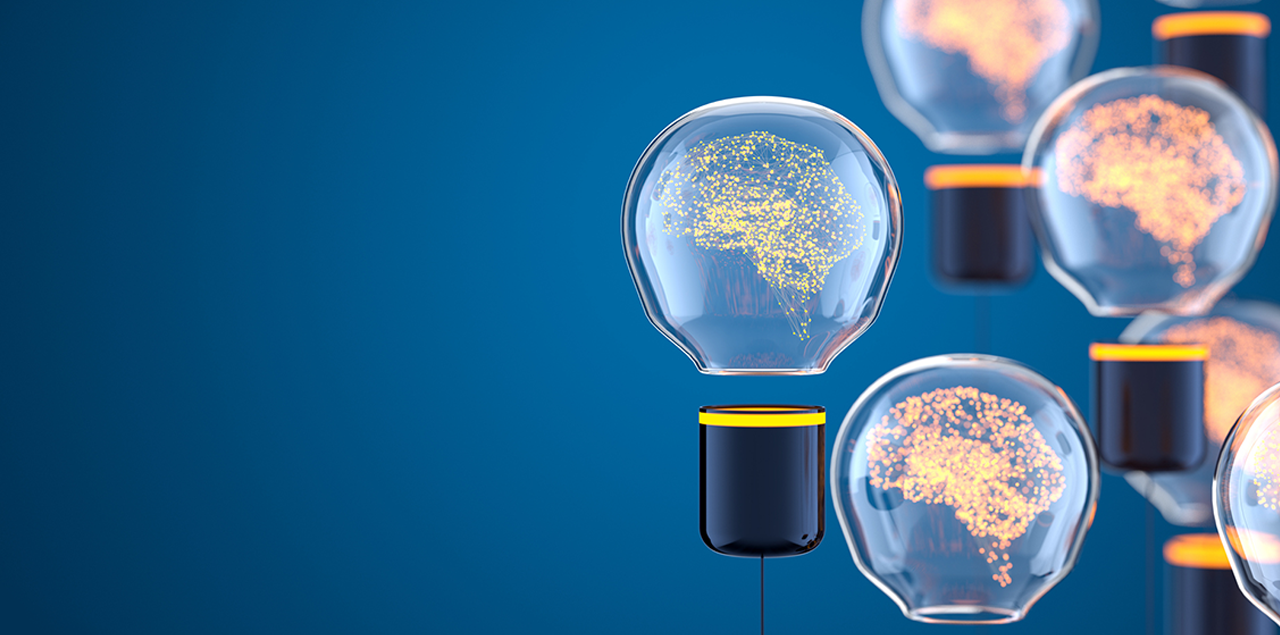
How to make sure machine learning projects succeed
More and more companies are looking to optimize processes, products and services with machine learning. The desire to introduce and invest is there, but there are still problems when it comes to implementing changes. Where do companies need to start if they are to rise to this challenge?
According to a recent study conducted by IDG, an average of two-thirds of German companies already use machine learning (ML) or are planning to introduce it; among large companies, the figure is as high as 73%. The study reveals that the need for ML is particularly evident in the IT sector, where increasingly complex infrastructures and ever more cyber-attacks cannot be managed efficiently without automated, self-learning systems. ML is also increasingly used in production environments, e.g., in process automation, predictive maintenance or supply chain optimization, the automation of B2C processes such as input management and in customer communication in the form of chatbots.
At first glance, it sounds promising that so many companies are now relying on ML. But what about the forecasts published by analysts such as Gartner that more than 80% of all ML and AI projects fail? Unfortunately, a look at everyday practice shows that this is still all too often the case. Just because a lot of companies use machine learning does not mean that they are successful with it. One reason for this is the quality of the input data. If it is not of high enough quality, there is no benefit. Furthermore, many employees and end customers lack confidence in AI-supported applications and services. If solutions are not developed to meet the particular requirements and do not deliver any noticeable added value, such reservations will be reinforced. Another challenge today is the heterogeneity of developer tools. The technology is still so new that there are no standards yet, and more applications are coming onto the market every day. The big question now is, how do companies that want to use machine learning successfully go about it? The following aspects can help them to achieve this:
1. Ensure data quality & quantity
Machine learning usually only works when there is a large enough amount of data with which to train the algorithms. Medium-sized companies in particular do not always have such pools of data at their disposal compared to large corporations. They need to top it up. One way to do this, for example, is to collaborate with partners from the same industry using federated learning. Here, ML models are trained within each company and then exchanged with those of other companies without the original data having to leave the organization. Companies that want to use their own data for machine learning would also be well advised to use a BI tool that helps with identifying and classifying the relevant data.
2. Define a use case roadmap
At present, it is easy to get the impression that almost every company wants to implement machine learning and artificial intelligence and is already taking the first steps in this direction. In fact, many projects have already passed the proof of concept, but they have not been implemented into business operations. For this to happen, it is advisable to identify the use cases that will be supported by ML at an early stage and to stagger them based on the expected success. Quick wins come first on the roadmap, while more complex use cases appear later. The quickly visible successes motivate and show how much potential can still be realized. This is also the time for trying out new things. Small failures can quickly be corrected at the beginning. These can then be learned from for further use cases without creating too much investment risk. Unfortunately, it is not possible to make a blanket statement about which use cases are particularly suitable and which ML tools are appropriate for implementing them. What counts here is empirical knowledge, which external consultants, for example, bring with them from previous projects they have worked on. Their neutral, external perspective also enables them to identify company-wide synergies that can easily be overlooked in individual projects.
3. Agile and step-by-step approach
Having a vision of a completely digitized, AI and data-driven company is great. It provides direction when it comes to reaching the next level in data-driven business. But who wants to put into practice a big picture that won’t have an impact for another ten years? Companies need solutions that will make a difference now. This is exactly what an agile approach makes possible. It pays to rely on small teams to achieve this. The important thing is that they include three different competencies: data science, technical and IT infrastructure expertise. ML solutions are developed based on the principles and methods of MLOps. This ensures that business and IT requirements as well as the conditions for operations to function smoothly are thought through together and implemented in a closely integrated manner that is always aligned to the corporate strategy and able to respond immediately to changing conditions. Such an approach yields quick wins and avoids lengthy projects that lead nowhere.
Machine learning needs the right mindset
Cloud infrastructure, monitoring, maintenance… There are many other aspects that are important if ML projects are to be successful. But above all, companies must not forget about cultural change. Machine learning not only requires new tools and specialists, it also changes the mindset in product development, in teams and in the entire company. Silo and departmental thinking often still get in the way of this. What is needed, by contrast, is a culture in which experimentation, continuous learning, and holistic, networked thinking and action become the norm.
Are you interested in machine learning and how it can be deployed in a business context? Dr. Lars Flöer and his colleagues would be happy to discuss this with you: you can get in touch with them here.