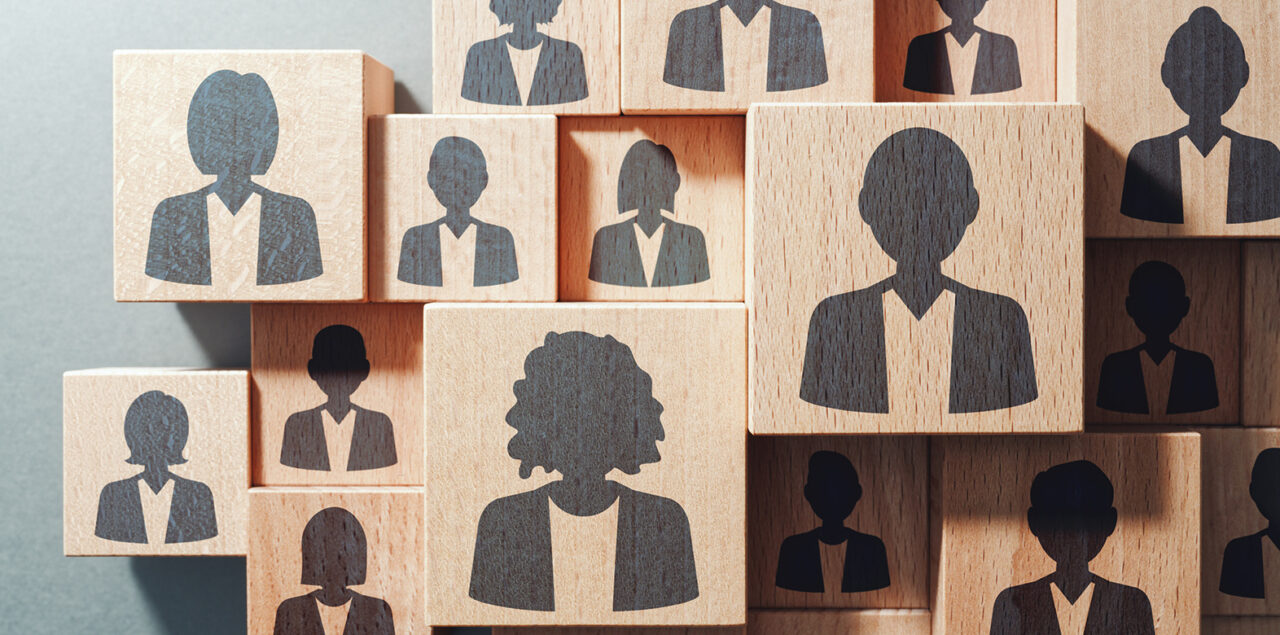
AI & Data Science: The roles and skills that companies need
An increasing number of companies are looking to become AI and data-driven - and are developing and strengthening the necessary skills in their teams. What roles and skills are necessary for this? And how do cross-functional teams work together successfully?
There has been a significant evolution in the level of sophistication in the use of analytics, machine learning (ML) and artificial intelligence (AI) in German companies in recent years. This is something we see every day in our customer projects. While a few years ago, proof of concepts and feasibility studies on the use of AI and analytics were still predominant, today machine learning and (generative) AI are often used operationally: from the automated processing of customer service inquiries to AI-based scrap control in the manufacturing industry and personalized healthcare.
Key roles in modern AI & data science teams
Data science and artificial intelligence are team sports. A broad spectrum of competencies and skills is required to successfully manage these often complex projects and to operate the necessary digital solutions in a stable and sustainable way. The teams should therefore include at least the following roles:
How team members work together optimally in data & AI projects
Besides the skills and roles described above, effective collaboration between data scientists and AI experts also requires all team members to work together seamlessly. This begins with brainstorming and continues right through to the implementation and maintenance of AI and analytics solutions. A typical workflow might look like this:
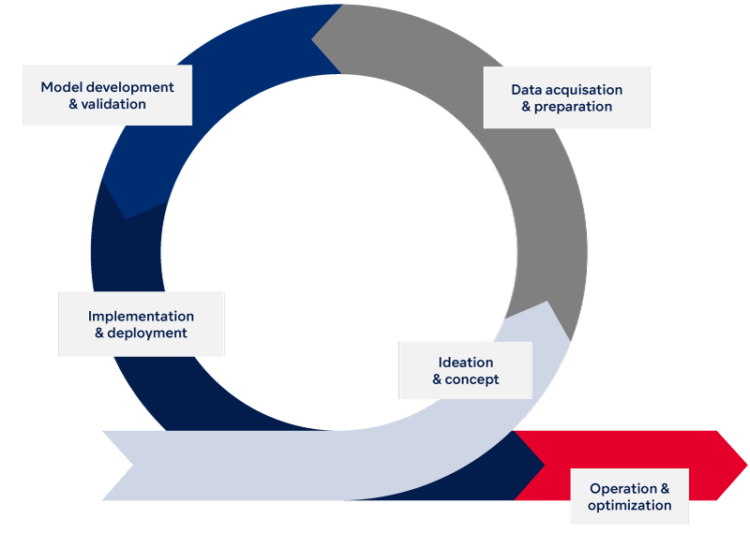
1. Brainstorming and concept
During this stage, the data strategists gather information about the company’s business goals and challenges. They conduct market analyses where necessary and identify opportunities to optimize and improve processes and products using data from a business process perspective. Together with the AI Specialists and MLOps Engineers, they work to develop concepts for data-driven solutions that support the achievement of business goals. These solutions are then to be implemented in the processes.
2. Data acquisition and pre-processing
The DataOps Specialists lead the way in this area. They identify and obtain the data required for modeling and operating AI solutions from various sources. They also undertake the necessary data cleansing and pre-processing to ensure that the data is suitable for modeling. Data quality and data availability are key challenges in almost all data projects.
3. Model development and validation
This is where the AI Specialists come into play again. They develop and then train the machine learning models using the data provided. Meanwhile, the MLOps Engineers work on the development of validation strategies for the performance of the mathematical models, i.e. they ensure that the model performance is evaluated.
4. Implementation and deployment
The ML Developers now take the lead. They integrate the models that have been developed into production environments and develop applications and interfaces via which the models can be addressed and used to improve business processes. The MLOps Engineers support the implementation of monitoring and maintenance processes to ensure that the models remain stable during operation.
5. Operation and optimization
The workflow does not end with implementation. The team continuously works on monitoring model performance and identifying opportunities for improvement. The MLOps Engineers play a central role in identifying potential areas for improvement in terms of model performance and infrastructure.
How to make sure machine learning projects succeed
More and more companies are looking to optimize processes, products and services with machine learning. The desire to introduce and invest is there, but there […]
Success factors and best practices for data & AI projects
In practice, clear communication, close coordination and ongoing training are important factors for successful collaboration. The team needs to fully understand the business objectives and the requirements of the various stakeholders both inside and outside the company, as well as being able to react flexibly to changes and ideally always being one step ahead. How does a data team become and remain successful? These are our lessons learned from numerous projects:
Clear communication and transparent cooperation:
Open communication between all team members is essential for the success of a data science team. Each team member needs to understand how their role contributes to the overall outcome and be able to openly discuss ideas and challenges. Regular meetings and status updates help to keep everyone involved up to speed.
Flexibility and adaptability:
The world of data is undergoing constant change, be it due to new technologies, changing business requirements or external influences such as changes in legislation. Successful data & AI teams are able to adapt quickly to new circumstances and react flexibly to changes. As a rule, they pursue an agile approach to work.
Continuous training and development:
In order to keep abreast of constantly evolving technologies and best practices, it is crucial that team members continuously expand their knowledge and skills. Further training measures, training courses and participation in conferences and workshops are therefore crucial for the long-term success of the team.
Stakeholder involvement:
Data projects are rarely isolated endeavors, but often influence various departments and interest groups within the company. It is therefore vital to involve all relevant stakeholders in the process from the outset, to understand their requirements and to ensure that the solutions developed meet their needs.
Clear objectives and measurability:
Every AI & data science project should have clear goals and KPIs that can be used to measure success. By setting common goals from the outset and regularly reviewing progress, the team can identify potential obstacles at an early stage and take countermeasures in good time.
If you would like to find out more about how to set up the data & AI team in your company effectively or if you would like support in certain roles, please contact Dr. Markus Knappitsch and his colleagues: You can contact us here.