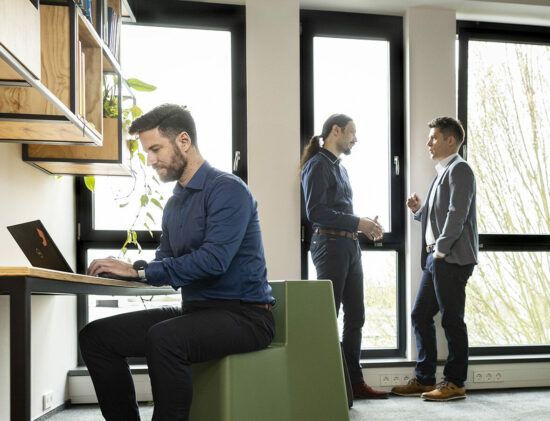
Data quality as a competitive advantage – an example from the pharmaceutical industry
In this era of data overload, it is vitally important for companies to manage their data effectively in order to remain competitive. Especially when it comes to vendor & service management, incorrect or duplicate data records (duplicates) pose an enormous risk. If, for example, transfers are made more than once or go to the wrong addressees, this results in unnecessary costs and delays. We know from projects with customers from the pharmaceutical industry that this can even delay their market entry. It may also lead to legal consequences if, for example, obligations to provide evidence of speakers are not fulfilled. Clean data management is therefore essential for competitiveness.
Duplicates as a data management challenge
Data management resembles a challenging team sport that requires coordinated interaction and perfect alignment between the stakeholders involved. Gigantic amounts of data generated in different regions and used by different systems frequently lead to a confusing data environment – full of incoherent and inconsistent information. Here’s a fictitious example: Prof. Dr. hc. Gertrude Belle Elion is listed as a duplicate by a pharmaceutical company in various systems and roles, including.
- hc. G. B. Elion, physician at the National Cancer Institute
- G. Belle Elion, professor at Duke University
- Trudy Elion, speaker at a HIV conference
In total, up to 10 different entries for different roles, organizational affiliations and addresses accumulate rapidly per person. When pharmaceutical companies have 500,000 or more system entries, you can imagine the chaos.
So how can this data landscape, which has often evolved over time, be cleaned up quickly and efficiently? And what to do to keep it then properly maintained?
The solution for efficient deduplication: AI & teamwork
Traditionally, deduplication is performed to clean up data via rule-based approaches that compare entries in the system on a pair-by-pair basis. These rules for comparison usually have to be created manually and are quite rigid. It becomes easier when the rule sets are created using an AI-based active learning approach. Here, an algorithm uses confirmed duplicates to learn which records are duplicates and can then identify them automatically. Even business logic is taken into account, so that “desired duplicates” can be distinguished from unnecessary ones. This approach also makes it possible not only to find duplicates and inactive data points, but also to make transparent partner networks and organizational structures in which data is created and used – the basis for continuously clean data management.
Since every organization’s data situation and business logic are different, there are no ready-made, off-the-shelf solutions for this AI-based deduplication. The key component in such projects, in addition to data science expertise, is therefore close collaboration with those who actually work with the data. The experts from vendor management and the servicing department check the quality of the results found with the help of AI at the start of the project and later in regular reviews. In this way, the ML-based solution can be aligned with the company’s individual processes and successively improved with each additional verified case. This ensures that the conditions of the company’s own data landscape are mapped and that local special cases are also correctly identified.
"In our master data alone, we were able to inactivate 58,000 suppliers whose data existed as duplicates or was outdated."
Frank Sommerer, Program Lead Master Data Quality, Boehringer Ingelheim GmbH
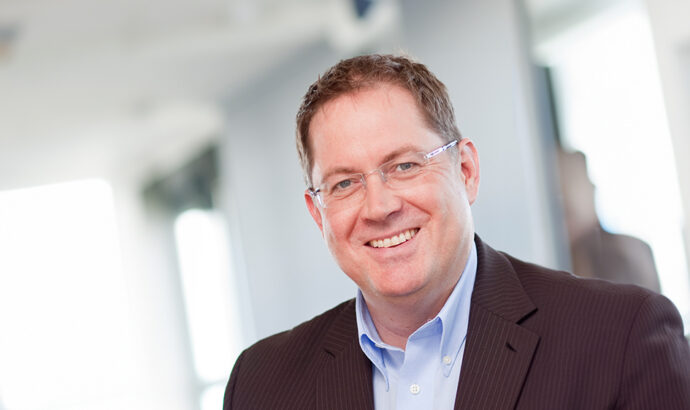
Staying ahead of the competition with AI
There are multiple benefits for pharma & life science companies from implementing AI-driven vendor & service management:
- Gigantic amounts of data become manageable.
- Transparency is created in vendor & service management.
- Controlling & reporting can be made more precise.
- Processes can be optimized on the basis of data.
- Compliance & regulatory risks are minimized.
- Cost efficiency increases measurably – and with it the ROI for the use of AI.
A further significant advantage is that errors and duplication of work resulting from the creation and maintenance of duplicate data records are avoided. This reduces the workload for employees and enables them to maintain the partner network more quickly and use it more efficiently.
Addressing data management strategically
Whether data is used within or between different teams: It takes a consistent, well-maintained base. To prevent the quality from declining in day-to-day operations, it must continue to be kept in good shape even after the “cleanup”. Data competence training is one way to help – because data quality starts with the input mask. Secondly, automation can avoid many mistakes. The manual transfer of data from one system to another, for example, can be automated. Vendor onboarding & background check or fraud detection can also be supported by AI and automated processes. A clean database is the foundation for this. At the same time, inconsistencies in these processes in turn become visible and can be cleaned up. Optimally, all these measures are implemented, interlinked, and continuously sharpened on the basis of an individual strategy – just like preparing for a match as part of a sports team.
Data management is a team sport. So why should you devote yourself to it as a lone wolf? If you are interested in the topic, feel free to exchange ideas with us!