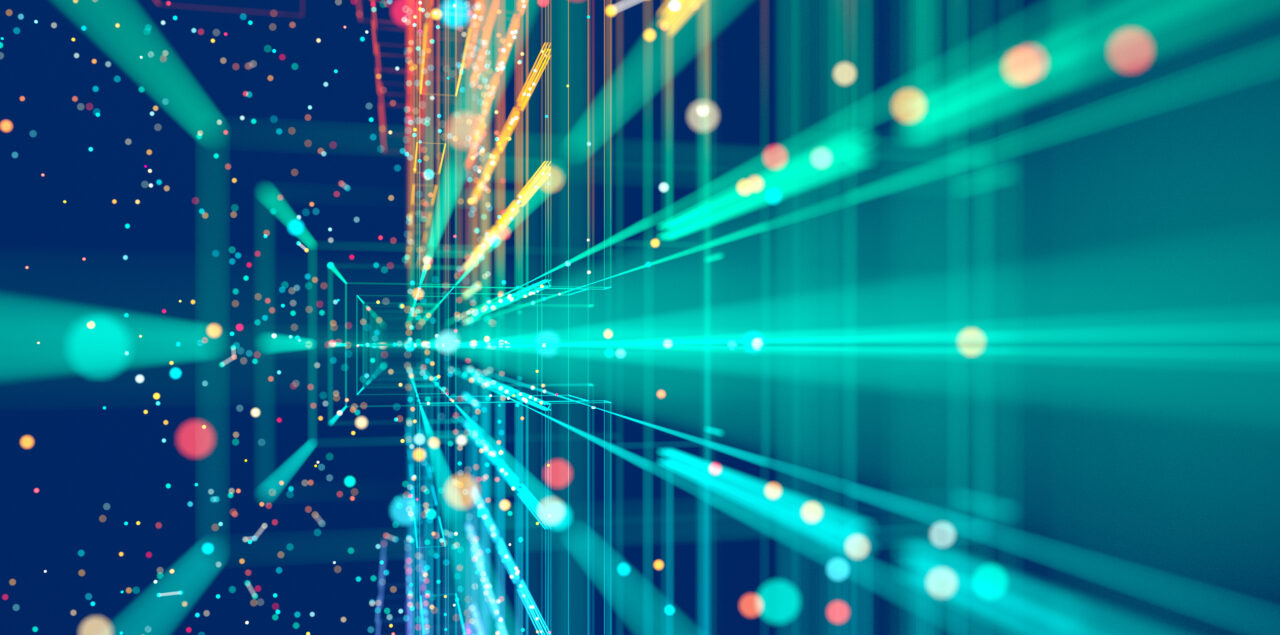
Data maturity at Boehringer Ingelheim: how AI is lifting data quality to a new level
Boehringer Ingelheim serves 130 markets around the world. Business both requires and generates massive quantities of master data - something that can only be handled and deliver added value when data management is 100% clean. To guarantee that the necessary data quality is achieved, Boehringer Ingelheim relies on the most cutting-edge AI techniques.
“As a globally operating corporation, we have a huge amount of data that has the potential to deliver important insights and enhanced functionality,” says Frank Sommerer. He holds the position of Program Lead Master Data Quality at Boehringer Ingelheim and, together with his team, is responsible, among other things, for ensuring that this complexity is made manageable. He therefore creates the foundation on which Boehringer Ingelheim can obtain data-driven insights and implement data-driven processes. A particular challenge lies in the management of central master data, which is used throughout the Group for all vendor and sales processes, and for other purposes.
“With a global corporation such as ours, the volume and complexity of our master data is continuously increasing. On top of this, there are also global and local compliance and regulatory requirements that need to be taken into account when managing master data. If errors occur here, the reputational and cost implications can be quite dramatic,” adds Frank Sommerer, explaining the significance of clean master data management. In order to minimize this risk and leverage data in an increasingly efficient way, Boehringer Ingelheim began looking for a solution.
In 1885, Albert Boehringer founded the pharmaceutical company Boehringer Ingelheim. From 28 employees back then, the number has grown to 53,000 today. The company operates globally in the human and biopharmaceutical and animal health sectors, serving more than 130 markets. Their global objective is to advance the health of people and animals.
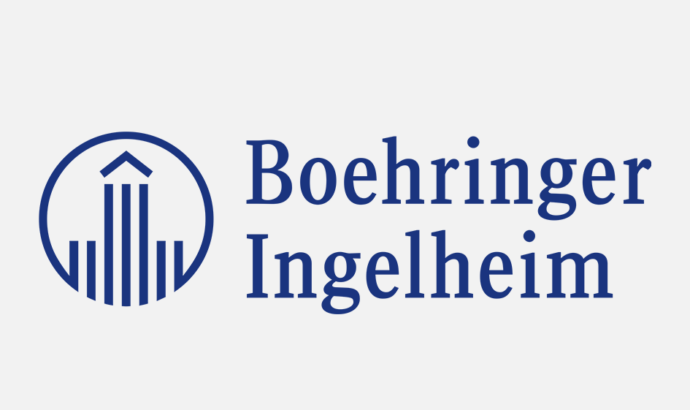
Data competence alone is not sufficient to create data quality
Many traditional approaches to data management, such as rule-based duplicate detection, lead to only marginal effects despite the high level of investment they require. Often, high-quality and comprehensive contextual information for making business-relevant decisions and optimizing processes is lacking. Added to this is the issue of data literacy. Employees must be trained to ensure data quality when creating new and maintaining existing master data. “Data quality is something that begins at the input mask. Training employees is therefore an essential step in improving data management,” says Frank Sommerer. He goes on to add, “No matter how well-trained people are, though, at some point they can no longer keep track of complex data volumes and need digital support. These are two factors we are working with Comma Soft to address.”
AI can be leveraged to master complexity
Artificial intelligence, especially in combination with graph theory-based approaches, is ideally suited to deal with the complexity described and to extract useful information from it. To achieve this, Boehringer Ingelheim first cataloged, analyzed and cleansed more than 400,000 existing data items with Comma Soft’s help. “In our master data alone, we were able to inactivate 58,000 suppliers whose data existed as duplicates or was outdated. Simultaneously, we also looked at the requirements that users have on a daily basis when working with this kind of data,” explains Frank Sommerer. These requirements range from quickly retrieving the data needed at any given time, through simple and correct data maintenance, to working with data sets that are subject to different regulations according to the respective region. To ensure that all of this works seamlessly, the processes were redesigned and focused on optimal data quality from the bottom up. Furthermore, Boehringer Ingelheim has established global standards (governance) that apply to the creation and maintenance of master data while at the same time taking country-specific ins and outs into account. In the future, a dedicated data quality dashboard will also ensure that Frank Sommerer and his team are able to see in real time what the current status of data quality is throughout the company and where they need to intervene and with what level of priority. “Consistent, correct, complete: these are the three key pillars to which we align data quality,” summarizes Frank Sommerer.
Data quality on the home straight
Data management is currently rolled out in almost all Boehringer Ingelheim divisions. Within the next two years, it is to become standard practice throughout the Group. “Instead of just optimizing data quality reactively in a worst-case scenario, as we used to do, we are now addressing it proactively – and taking big steps towards the ‘managed’ level, where we can control it consistently and cost-effectively,” Frank Sommerer asserts confidently.
Do you have any thoughts or questions about data quality and data management? Please feel free to contact Dr. Henning Dickten and his colleagues directly: you can get in touch with them here.