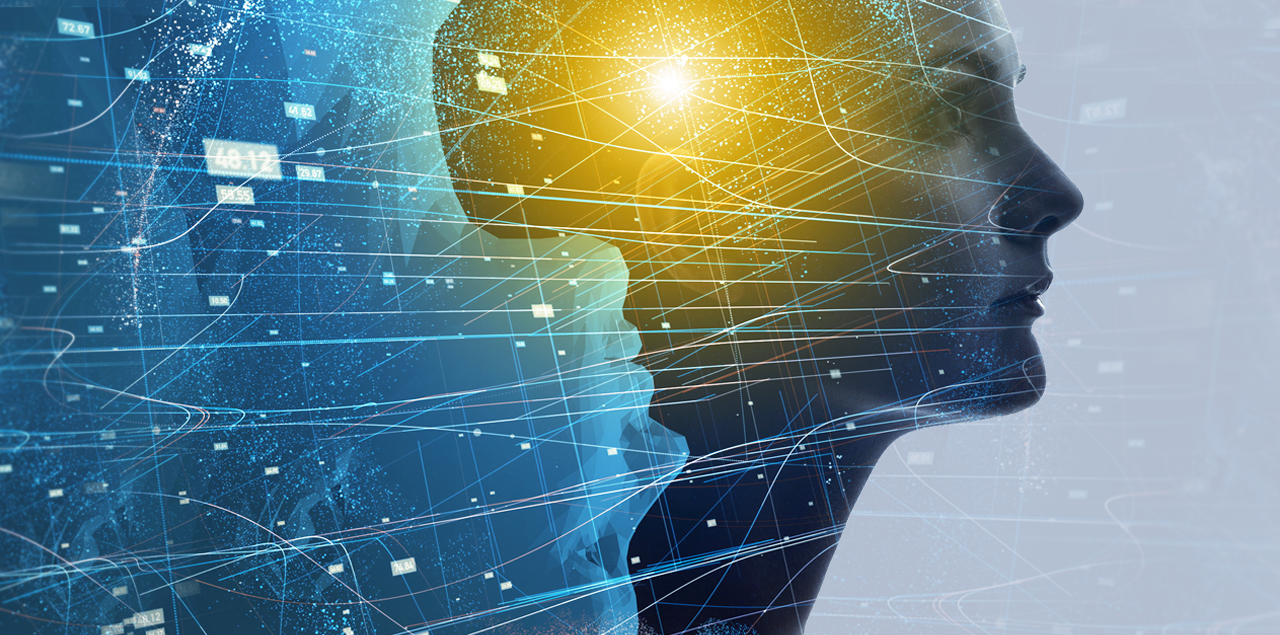
Personalized medicine: digital twins revolutionize healthcare
Faster drug approval, personalized treatment, improved prevention: there is enormous potential for research and healthcare in digital twins. Dr. Henning Dickten, Head of Consulting Pharma & Life Science at Comma Soft, explains in which fields of application they can be used, how compliance hurdles can be overcome using federated learning, and what role a data & AI strategy plays in this.
Digital twins that use data and algorithms to simulate the status, functions and reactions of machines and processes, among other things, have been a familiar sight in industry for a long time now. This enables industrial companies to develop prototypes quickly and cost-effectively and to streamline their entire value chain. Digital twins also offer numerous advantages in medicine and pharmaceutical research: trial cohorts can be created with virtual patients, which accelerates drug development. Doctors can make diagnoses more quickly and simulate therapies on digital twins. This results in better opportunities for personalized medicine and lower healthcare costs. Nevertheless, only limited use of digital twins in medicine and pharmaceuticals has been made to date. What is the reason for this and how can existing hurdles be overcome?
No digital twin without data
Digital twins are simply built on data – lots and lots of high-quality data. They are first used to create general models that emulate physiology or the way people respond to medication, for example. In the second step, this generic model is used to create a digital twin that is tailored to the individual patient. It makes it possible to predict the course of a treatment and alternative therapies before they are applied to the patients themselves. Multiple such digital twins can then be combined into virtual test cohorts and used for studies of treatment protocols and outcomes, saving both time and money. The big question is: from where do pharmaceutical companies, research institutions and hospitals get the necessary data? Treatments and examinations that have actually taken place are one source, and another could be research studies that have been set up specifically for this purpose. However, these volumes of data collected at the respective institutions are usually insufficient for efficient use of digital twins, especially in the case of rare diseases. If institutions were to network with each other and share their data, the situation would be very different. This is exactly where the problem lies in practice: regulatory requirements impede the exchange of data. As a result, each organization keeps its treasure trove of data to itself. It doesn’t have to stay that way, and it shouldn’t.
Sharing health data securely with federated learning
Simply sharing sensitive personal health data is not legally or ethically defensible. Federated machine learning approaches that make share-without-sharing possible offer a safe alternative here. The data of patients and trial subjects stay in the respective organization. Only the generalized insights generated by their digital twins are shared. These results can be used to train and improve digital twins individually at other facilities without using the original health data. There are no data privacy issues and no data are wasted. By exchanging information across the boundaries of pharmaceutical companies, hospitals and even service providers, the entire healthcare value chain can then be improved – using digital twins and numerous other digital healthcare services:
- The pharmaceutical industry can speed up clinical trials and bring drugs to market more quickly.
- Health insurers use digital twins for preventive services, e.g., in health apps, and thus reduce cost-intensive treatments.
- Physicians can improve early detection of dementia and depression, for example, and mitigate their progression.
- Hospitals offer personalized therapies, for instance for osteoporosis, cancer, or metabolic diseases, which respond more effectively and more quickly.
- In medical training, students use digital twins to gain hands-on experience before treating real people.
This presents an opportunity to integrate prevention, early detection, treatment, follow-up, and teaching. Alongside the positive effect on healthcare costs, extensive use of digital twins would improve the health of numerous people in the medium term, thereby enhancing their quality of life and productivity.
Making IT infrastructure fit for federated learning
New technologies like federated learning overcome compliance hurdles. But they also bring with them a challenge: the IT landscape must be designed for data and AI-driven processes as well as capable of sharing the research findings in a secure manner. How well is the infrastructure set up for this, and what know-how does the company’s own IT department bring to the table? Would it be worthwhile to have a cloud strategy or to connect to an external collaboration platform? What interfaces will this require? These questions are often answered by external experts who have experience in similar projects and can provide support not only with modernizing the infrastructure but also with implementing and deploying machine learning. At the same time, external consultants can act as a link between business units and IT, ensure close dovetailing between all units and promote the creation of synergies. Dr. Henning Dickten and his team have already guided numerous organizations on their way to becoming data-driven companies by adopting this approach. They will also be happy to lend you a helping hand with their expertise. If you would like to find out more, you can get in touch with them here.