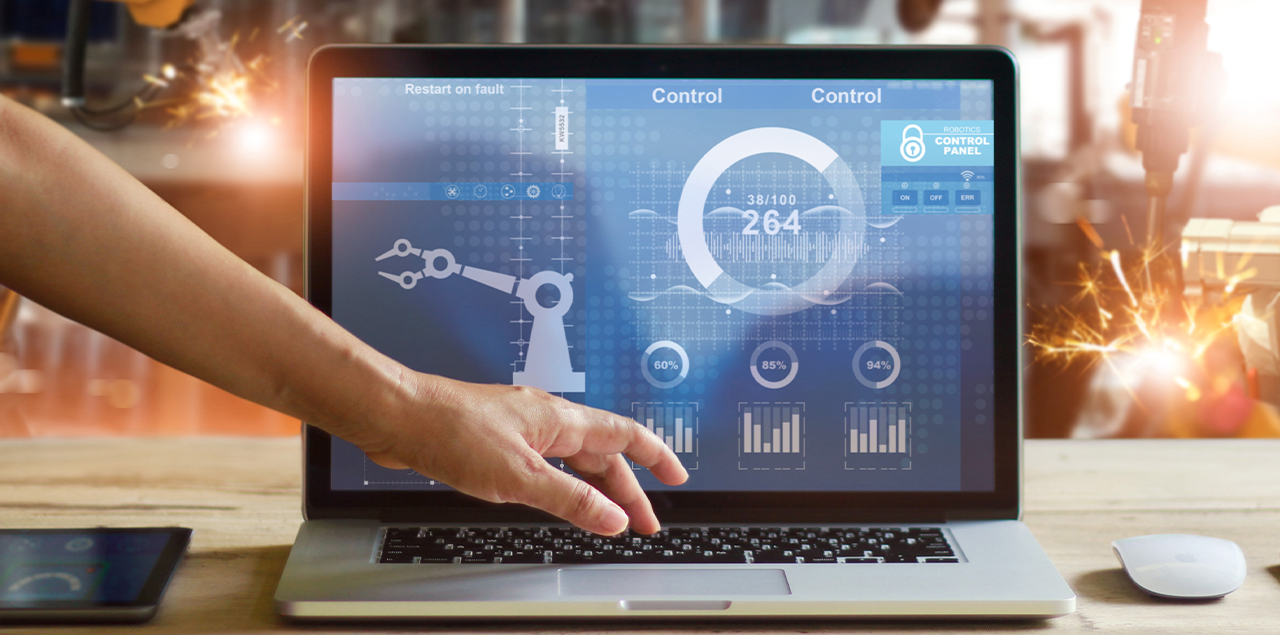
Expert Talk: “For manufacturing companies, there’s no way around data and AI in these tenuous times”
Sustainability, new reporting obligations, fragile supply chains: Manufacturers are currently facing an increasing number of new challenges. Our industrial IoT expert Dr. Oliver Beyer explains in an interview how you can use your data and (generative) AI to quickly and sustainably position yourself as a competitive player.
Oliver, you have been active in the manufacturing industry for many years, and as a member of various AI and Industry 4.0-related specialist committees, you maintain an ongoing and close exchange with industry. What issues are currently on companies’ minds?
Right now, the main omnipresent topics are bolstering the resilience of supply chains, improving energy efficiency, and reducing CO2 emissions for more sustainable production. Drivers here include the current geopolitical disputes, Germany-wide regulations such as the Supply Chain Act (LkSG) and also the EU’s CSRD Directive. For companies, this means that they need to digitally map and make available material flows across the entire supply chain, optimize their own production from an energy perspective through process optimization and the use of renewable energies, and pinpoint ESG-relevant data such as their carbon footprint. The challenge here is not only to make the relevant data accessible, but also to make it available on a cross-company basis. An exchange, for example, requires open, standardized and trustworthy data ecosystems such as Catena-X and data formats such as the Industrial Digital Twin Association’s Asset Administration Shell (IDTA) in order to create the appropriate basis for data-driven and resilient value chains.
As the use of AI becomes increasingly relevant, the demand for transparency and human-centered application is in the foreground, especially when it comes to production. Here, it is important to create trust and dispel fears by applying methods such as “ExplainableAI” (XAI). The ubiquitous implementation of AI solutions, e.g., through edge computing in the IoT context and operationalization via MLOps, also present major challenges, especially for medium-sized companies.
Terms like IoT or Industry 4.0 are now well over a decade old. Have the topics of digitization and AI become a bit old hat?
Not at all. For both the national market and international competition with the USA and China, digitization is a fundamental factor that enables German and European companies to remain competitive. This is the only way they can keep up with the fully digitized and cost-optimized production processes of their competitors. There is a clear consensus among companies on this: digitization and the use of AI are still far from being sufficiently implemented and will have a lasting impact on industry in the future. Especially in these uncertain times and in the face of increasing complexity, one thing is clear: the need for greater focus on data and AI is essential.
More specifically, the focus here is both on the resulting potential for increasing production efficiency, such as raising overall equipment efficiency (OEE), and on reducing costs through more sustainable and energy-efficient production processes. At the same time, the focus is on developing new digital business models, which will play an increasingly important role in the future. Here, a trend toward customer-centric digital services is emerging, for example using apps to offer customers greater transparency about manufacturing and logistics processes, more individual product configurations, or new services. The aim here is to simplify business processes on the customer side, establish new services and thereby increase customer loyalty.
The use of AI and generative AI is an important driver and essential for many of the targeted use cases, both in increasing production efficiency and in opening up new digital business models.
Which AI cases do companies specifically want to implement and how do they go about it?
The answer is multifaceted. From forecasting orders and material flows to optimizing production parameters and delivery & sales planning to recommending systems for customer ordering processes, everything is included.
There are, however, significant differences in implementation. While some companies have already digitized and integrated some processes along the value chain, others are still at the beginning of their digital journey. Indeed, some are skeptical about the use of AI solutions as part of their production processes. After the first initial proof-of-concepts, which can be implemented as lighthouse projects, e.g., in production for machine or process monitoring, there is often an initial disillusionment. With selective cases, it is not possible to achieve as much efficiency improvement as would be desirable.
What might an alternative to such selective cases look like?
The best approach is to integrate AI into business processes in a sustainable manner and to use it consistently across all areas – the key phrase here is: data-driven end-to-end processes. But in order for AI to be made effective along the entire value chain, it must be given sufficient and high-quality data. This requires an end-to-end strategy for using the company’s own data and – in the context of supply chain transparency – for incorporating external data.
Many companies are still hesitant here, especially when it comes to providing production-related data. The issue of information security is again more topical than ever, not least due to ChatGPT and other generative AI solutions. If customers and suppliers are to be included in processes, for example, this is absolutely essential. The fear that this would mean that important knowledge and information on production processes and capacity utilization would also become available to competitors is great. The development of trustworthy data ecosystems such as Catena-X, mentioned above, is currently creating a new perspective in this regard though.
Digital ethics: What does it mean?
Digital ethics, also called AI ethics when focusing on AI, is the buzzword of the day. But what is covered by this term? Why is […]
How do you and your colleagues support companies in this?
For one thing, we help with the development of the data strategy, e.g., with the aid of data maps. This already creates a great deal of security and helps prevent digitization from turning into an incalculable financial investment for companies. Instead of implementing isolated digitization projects “on the side” with often semi-optimal results, a data strategy helps to maintain an overview right from the start and to plan sufficient resources. This not only includes the budget for new technologies, but also IT and data science expertise to estimate expenditure and implement the necessary architectural structures, evaluations and, ultimately, cases. Especially in the field of generative AI, we also work with our customers to develop individual solutions that ensure data protection and information security. If the IT and data science capacities in the company are not sufficient for all of this, we also offer our support here.
Besides the new technologies, it is also important to ensure that staff are sufficiently involved. What specific support do they need in their daily work? Within the framework of change management, we support companies in building trust in new technologies among employees, in developing a common basic understanding, and then in jointly implementing solutions that are actually used and create added value. The increasing lack of skilled workers in production facilities further reinforces the need to support people with shop-floor-related data-driven assistance systems, e.g., for machine operation and in the manual workplace.
This kind of strategic approach sounds lengthy. Is there also a way to achieve quicker results?
A tried and tested approach is the dual approach involving a data strategy on the one hand and the pragmatic implementation of use cases with little effort and a major impact on the other. The cases should not be used in isolation, however, but should be embedded in a holistic strategy from the outset. This strategy can then take a very different form depending on the company’s level of digitization. While for some companies the biggest challenge is data availability and breaking down data silos, for others it’s automating and integrating business processes, or data-driven forecasting of orders or inventory levels. The precise levers are usually highly individual and are identified by us together with the companies. Similarly, we also jointly prioritize which cases can be implemented promptly and with manageable effort, and which are more suitable for later on. All in all, this then results in a good mix of quick wins and sustainable solutions with which companies can position themselves competitively now and in the future.
What challenges are you currently facing in your company? Please feel free to discuss this with Dr. Oliver Beyer and his colleagues: You can get in touch with them here.