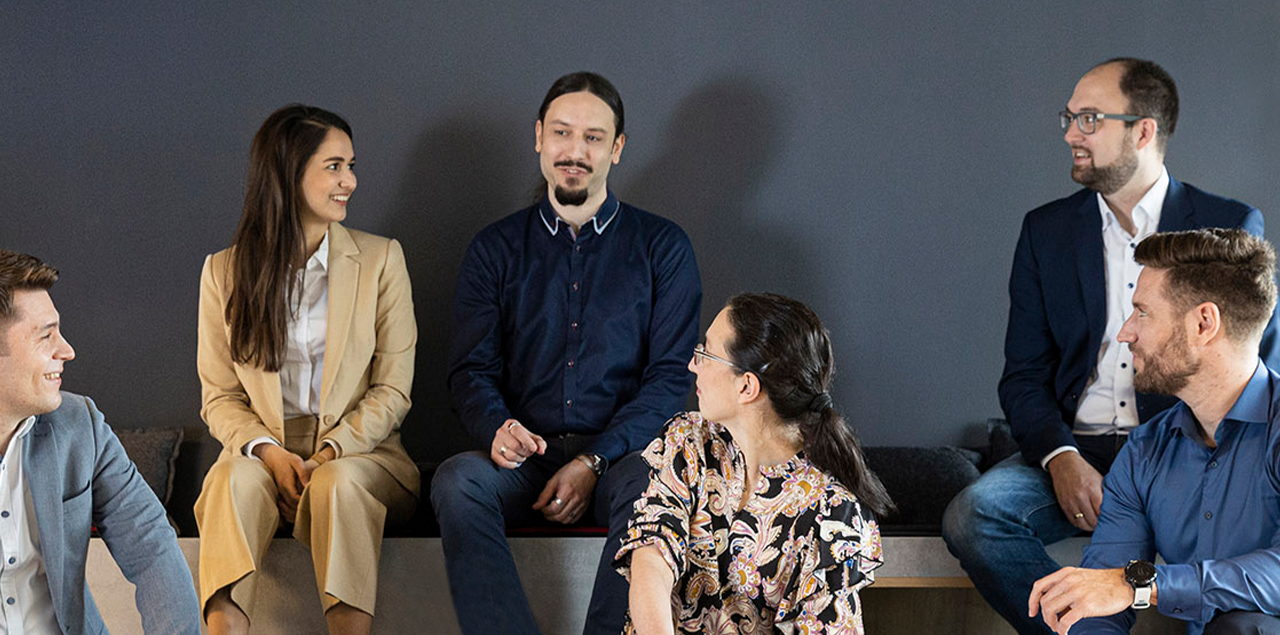
Pharma Expert Talk: “We use data to make the world just that little bit better!”
The pharmaceutical industry is currently undergoing a realignment: moving away from consolidation to partnerships with external AI & data science experts. In this interview, Dr. Henning Dickten reports on how these endeavors are not only contributing to entrepreneurial success but also to assuming social responsibility.
Judging by the media, it would appear that the pharmaceutical market is currently at a turning point. What is currently occupying the industry?
In the past few years, there has been a lot of focus on mergers and acquisitions of smaller companies with a view to buying in expertise on a large scale. Currently, we see that this trend is flattening out. Instead, the larger companies are stepping up their efforts to build up AI expertise in a more targeted manner. To this end, there are many internal training programs, but also many additional strategic partnerships with start-ups and consultancies. One way they do this is by bringing in external data science expertise. This enables them to respond relatively quickly to new technological trends such as (generative) artificial intelligence and to expand their offerings towards personalized medicine and patient empowerment without having to first build up their own resources.
Other key topics include sustainability, resilient supply chains and the use of e-health platforms to connect patients, trial participants, physicians and other healthcare stakeholders. On balance, it can be said that the focus is shifting from consolidation to partnerships and that social responsibility is being taken more seriously.
Expert Talk: “efficient production alone is not a measure of sustainability!”
Today, sustainability is more than just a trendy nice-to-have: it is demanded by politicians, financial markets and customers and is a decisive criterion for competitiveness. […]
What new challenges does this pose for pharmaceutical companies?
Currently, I see four main issues for pharmaceutical companies. Firstly, a high degree of personalization of medicines and medical products and a simultaneous increased focus on the customer. To achieve this, the companies naturally need the relevant data from the patients. The question is, where do you get them and at the same time comply with regulatory requirements for data protection? The German Health Data Use Act (GDNG) aims to make this possible. From a technical point of view, the whole thing can be approached with digital twins and federated learning.
Secondly, the “time-to-market“. The goal is to make new active ingredients and treatments available more quickly – e.g., through predictions using AI and data. This makes it possible, for example, to shorten the development of a drug from 12 years to less than 10 years – which is still a considerable period of time, but in many cases faster than a lot of competitors.
The third focus is on usability and data quality across the entire value chain. It is often the case that data is still collected selectively, e.g., for individual projects or individual experiments. These individual data sets, however, do not result in a usable overall data set due to a lack of standardization. Yet this is necessary, for instance, to model and automate processes from A to Z and to make valid forecasts whenever required. This then enables data-driven decisions to be made at every point in the value chain – from R&D to production to sales.
Simultaneously, it is important to keep in mind that such a focus on data-driven processes also requires a trained approach to data – the keyword here being “data competence”. In this context, it is important to involve employees at an early stage and to train them accordingly, from the administrative staff in customer service through to the specialist departments all the way to management.
And finally, the fourth aspect is the modernization of the entire IT infrastructure, through which analyses, data use and process digitization take place. This is where access to data or AI-trained algorithms is still an issue. I was delighted that we were able to contribute to the ImmunoHub project aimed at researching COVID-19 by using federated learning approaches and suitable infrastructure to help ease the burden here. Such solutions, however, are yet to become more widely established.
The orientation toward partnerships, through which technological and organizational change is accompanied and accelerated , is in my view exactly the kind of approach that pharmaceutical companies can use to address these four points.
What specifically should pharmaceutical companies be addressing together with their partners?
Driving the digital transformation forward. This may sound like old hat, but it is far from being exhausted everywhere. There are still many sources of friction on the way from the discovery of a potential drug candidate to a market-ready product: designing suitable studies is often mapped with complicated Excel spreadsheets, recruiting suitable cohorts with email drops, conducting studies and close-outs are done in-house (mostly project-specific) databases and the respective problems and findings are not fed back. All these steps can often be modeled by a consistent digital thread using all the available and relevant information, modern systems and suitable processes, and can be significantly accelerated and streamlined with AI assistants.
Given that companies are currently thinking more in terms of networks, partnerships and collaborations, they can tackle such issues well without overextending themselves. In the field of AI and IT infrastructure, many of our customers are now asking themselves the following sensible question: “Is this our core competency, or could we not benefit from the expertise of others and call on their services as needed?” After all, I wouldn’t develop medicines myself, but focus instead on my specialty: data science. My team and I can use this science to help pharmaceutical companies.
Why have you and your team placed the focus on pharma & life science in particular?
First of all, I must emphasize that my colleagues and I at Comma Soft work in a wide variety of industries. Everything from the insurance industry through manufacturing right up to pharmaceuticals and life sciences. Many of them also conducted research and earned doctorates in medical fields prior to working at Comma Soft, such as neuro-oncology, epileptology, or medical imaging. The latter has a special significance for us, since the majority of us come from the scientific community. In our team, you will find people with backgrounds in mathematics, physics, chemistry and biology as well as in the humanities. What we all have in common? We don’t just want to research in theory, we want to put solutions into practice in the real world. Building the “bridge between science and business,” as we say. This is why pharmaceutical and life science companies, with their high focus on R&D, are of particular interest to me. I also see a profound value in pharma & life science, for instance when drugs are developed that save lives, curb diseases like COVID-19, and thus help people in a very tangible way. So, with data and algorithms, my team and I can help make the world a little better every day.
How specifically do you and your team make a contribution to a “better world”?
There are lots of possibilities. Together with our partners, we are working on ways to improve the diagnosis and treatment of diseases, among other things. For example, as part of studies such as those on the lung disease COPD, in the mapping of the lungs in the Human Lung Cell Atlas project, or as part of the project on COVID-19 therapies, in which we implemented the technological platform for the exchange of research data and findings with our partners.
We are also exploring how to improve the work in pharmaceutical companies themselves. For instance, we used machine learning to clean up duplicates in the global CRM system used by a pharmaceutical company. This facilitates the work of the employees considerably, and it is great to see the relief evident on their faces. Simultaneously, the clean database is essential for collaboration with organizations and researchers around the world. Another example can be found in the production process of crimping. With the help of machine learning, we were able to identify key drivers and derive a formula that enables predictions for test runs. This shortens these test runs substantially – production lines do not have to come to a standstill and drugs can thus be manufactured and used to treat people much more quickly. These are just two examples of the many exciting projects that are close to my heart.
In other words, pharmaceutical companies can no longer do without data and AI. What are your thoughts on generative AI like ChatGPT? Will it also be used in pharmaceutical companies in the future?
That’s a very good question – I can definitely see possibilities here. In theory, generative AI can assist in writing applications and reports for government agencies, product information, or even drug package inserts or scientific articles. It is possible to generate target group-oriented texts that make information accessible to both healthcare professionals and laypersons. However, in a highly regulated industry like pharma & life science, the question of digital ethics, data protection and compliance must always be considered. This is still a hurdle for which every company must find its own solution.
In addition to generative AI, however, there are already numerous “classic” AI solutions that can be safely used in a company’s operations . Image recognition and the analysis of X-rays or MRI scans is one example of how AI is already being used in healthcare and can also support pharmaceutical research when anomalies need to be identified. This means that there is no need to decide for or against ChatGPT and similar tools. Ultimately, it is more important to achieve your goals by using the technology that is right for you. Like a jigsaw puzzle, you have to find the right piece and then you are rewarded with the big picture – with no two pictures being the same. That’s what makes my work so exciting.
If you would like to further discuss current trends and challenges in pharma & life science, please feel free to contact Dr. Henning Dickten and his colleagues: You can get in touch with us here.